Defining Business Intelligence in Supply Chain Context
Business intelligence solutions for supply chain optimization – Business intelligence (BI) in supply chain management is the process of collecting, analyzing, and interpreting data to gain actionable insights that improve efficiency, reduce costs, and enhance overall performance. It moves beyond simple reporting, providing a strategic advantage by illuminating hidden patterns and trends within the complex web of supply chain operations. Think of it as a powerful lens that clarifies the often-murky waters of inventory management, logistics, and procurement.
A robust BI system for supply chain management integrates data from various sources – enterprise resource planning (ERP) systems, customer relationship management (CRM) systems, warehouse management systems (WMS), transportation management systems (TMS), and even external data sources like market trends and weather forecasts. This integrated view is crucial for holistic understanding and effective decision-making. The system then processes this raw data, applying analytical techniques to identify areas for improvement, predict future trends, and support proactive interventions.
Core Components of a Supply Chain BI System
A comprehensive BI system for supply chain optimization comprises several key components working in concert. These include data warehousing to consolidate data from diverse sources; data integration tools to harmonize and cleanse data; data mining and analytical tools to uncover patterns and insights; reporting and visualization dashboards to present findings clearly and concisely; and finally, a user-friendly interface allowing seamless access and interaction for all relevant stakeholders.
The interplay of these components is what empowers effective supply chain management.
Examine how building a successful business intelligence team and culture can boost performance in your area.
Key Performance Indicators (KPIs) in Supply Chain BI
Effective supply chain BI relies on tracking a set of crucial KPIs. These metrics provide a quantifiable measure of performance across various aspects of the supply chain. Some key examples include inventory turnover rate, which measures how efficiently inventory is managed; order fulfillment cycle time, reflecting the speed and efficiency of order processing; on-time delivery rate, indicating the reliability of delivery; and logistics costs as a percentage of revenue, showing the efficiency of logistics operations.
By closely monitoring these KPIs, companies can pinpoint areas needing improvement and track the impact of implemented changes.
Real-Time Data Visualization in Supply Chain Decision-Making
Real-time data visualization significantly enhances supply chain decision-making. Imagine a dashboard displaying live updates on inventory levels across multiple warehouses, coupled with real-time tracking of shipments. This immediate visibility allows for proactive responses to potential disruptions, such as adjusting inventory levels to prevent stockouts or rerouting shipments to avoid delays caused by unforeseen circumstances, like severe weather. For example, a sudden surge in demand for a particular product can be immediately identified, enabling the company to quickly adjust production schedules and allocate resources accordingly, minimizing lost sales opportunities.
This proactive approach, empowered by real-time data visualization, transforms reactive problem-solving into strategic, anticipatory management.
Comparison of BI Software Features for Supply Chain Optimization
Feature | Software A | Software B | Software C |
---|---|---|---|
Data Integration Capabilities | Supports various data sources, including ERP, CRM, WMS | Limited to specific ERP systems | Broad integration with various cloud-based platforms |
Real-time Data Visualization | Interactive dashboards with customizable views | Static reports with limited real-time capabilities | Advanced visualization tools with predictive analytics |
Predictive Analytics | Basic forecasting models | Advanced machine learning algorithms for demand forecasting | Integration with external market data for improved forecasting |
Reporting and Alerting | Customizable reports and email alerts | Pre-defined reports with limited customization | Automated alerts based on predefined thresholds and customizable rules |
Data Sources and Integration for Supply Chain BI
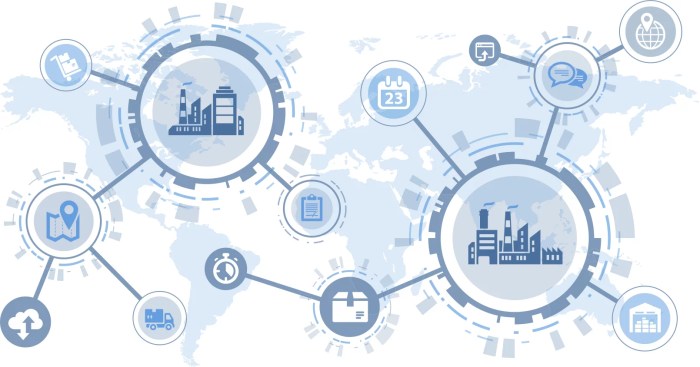
Unlocking the true potential of Business Intelligence (BI) in supply chain management hinges on effectively harnessing data from diverse sources. A robust BI system isn’t just about collecting data; it’s about integrating, cleaning, and analyzing it to gain actionable insights that drive efficiency and profitability. This involves understanding the various data sources, tackling the challenges of integration, and implementing strategies to maintain data quality.Data Sources Used in Supply Chain BI represent a multifaceted landscape.
Each source offers unique perspectives on the supply chain’s performance, and their combined power is what truly enables comprehensive analysis.
Key Data Sources in Supply Chain BI
Supply chain BI draws data from a variety of internal and external sources. Effective integration of these sources is crucial for creating a holistic view of the supply chain. Common sources include Enterprise Resource Planning (ERP) systems, Customer Relationship Management (CRM) systems, Warehouse Management Systems (WMS), Transportation Management Systems (TMS), and even external sources like market data providers and weather services.
ERP systems provide data on production, inventory, and financials; CRM systems offer insights into customer behavior and demand; WMS track inventory movements within warehouses; TMS manage transportation logistics; while external sources offer context beyond the immediate operational environment. The integration of these diverse data streams is key to achieving a unified and comprehensive understanding of the entire supply chain.
Challenges of Integrating Disparate Data Sources
Integrating data from various sources presents significant hurdles. Data inconsistencies, differing formats, and the sheer volume of data can make integration a complex and time-consuming process. For instance, discrepancies in product codes across different systems can lead to inaccurate inventory counts. Real-time data synchronization across multiple systems can also be challenging, potentially resulting in delays in decision-making.
Furthermore, ensuring data security and compliance with regulations adds another layer of complexity to the integration process. Overcoming these challenges requires careful planning, robust data governance, and the use of appropriate integration technologies.
Strategies for Ensuring Data Quality and Accuracy
Maintaining data quality is paramount for the reliability of supply chain BI. This involves implementing data cleansing, validation, and standardization procedures. Data cleansing involves identifying and correcting inconsistencies, such as missing values or duplicate entries. Data validation ensures that data conforms to predefined rules and constraints. Standardization involves converting data into a consistent format to facilitate seamless integration across different systems.
Regular data audits and quality checks are essential to proactively identify and address potential issues. Employing data governance frameworks and establishing clear data ownership responsibilities further enhance data quality and accuracy. Investing in data quality tools and technologies can automate many of these processes, improving efficiency and reducing the risk of errors.
Example Data Integration Architecture
Imagine a hypothetical supply chain for a large consumer goods manufacturer. Their data integration architecture could leverage an enterprise service bus (ESB) as a central hub. The ESB would connect to various data sources, including an ERP system (SAP), a CRM system (Salesforce), a WMS (Manhattan Associates), and a TMS (Oracle Transportation Management). Data from these sources would be transformed and standardized using ETL (Extract, Transform, Load) processes before being loaded into a data warehouse.
The data warehouse would then serve as the central repository for BI reporting and analytics. This architecture allows for real-time data integration and ensures data consistency across different systems. The ESB’s ability to handle various data formats and protocols simplifies the integration process, while the data warehouse provides a centralized location for analysis and reporting. Regular data quality checks and monitoring mechanisms would be implemented to ensure the accuracy and reliability of the data.
This comprehensive approach ensures that the BI system provides accurate and timely insights, enabling data-driven decision-making across the entire supply chain.
Predictive Analytics for Supply Chain Optimization
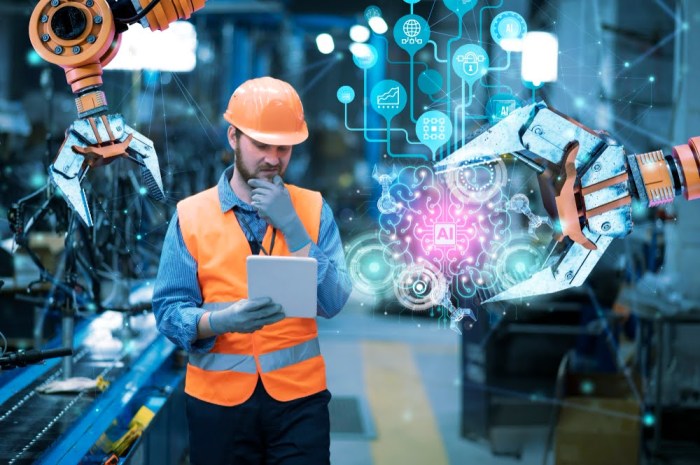
Predictive analytics is revolutionizing supply chain management, moving beyond reactive problem-solving to proactive, data-driven decision-making. By leveraging historical data and advanced algorithms, businesses can anticipate future trends, optimize resource allocation, and mitigate potential disruptions before they impact the bottom line. This empowers companies to achieve greater efficiency, reduce costs, and enhance customer satisfaction.Predictive analytics techniques offer a powerful arsenal for improving supply chain performance.
These techniques analyze vast datasets to identify patterns and predict future outcomes, allowing businesses to make informed decisions about everything from inventory management to transportation logistics.
Demand Forecasting Techniques
Several predictive analytics techniques are used to forecast demand and optimize inventory levels. Time series analysis, for example, uses historical sales data to identify trends and seasonality, projecting future demand based on these patterns. Regression analysis explores the relationship between demand and various factors like price, promotions, and economic indicators, creating a model to predict demand under different conditions.
Machine learning algorithms, such as ARIMA (Autoregressive Integrated Moving Average) and Exponential Smoothing, are particularly effective in handling complex patterns and noisy data, providing more accurate and robust forecasts. For instance, a retailer might use ARIMA to predict seasonal fluctuations in demand for winter coats, allowing them to optimize their inventory levels and avoid stockouts or excess inventory.
Machine Learning Algorithms for Supply Chain Efficiency
Machine learning algorithms are transforming supply chain efficiency. These algorithms can identify complex patterns and relationships in data that are often invisible to traditional methods. For example, anomaly detection algorithms can identify unusual patterns in delivery times or supplier performance, flagging potential disruptions early on. Clustering algorithms can group similar products or customers, enabling targeted inventory management and optimized delivery routes.
Reinforcement learning algorithms can even automate decision-making processes, such as optimizing warehouse layout or dynamically adjusting transportation routes in response to real-time conditions. Imagine a logistics company using reinforcement learning to optimize delivery routes in real-time, accounting for traffic congestion and weather conditions, leading to faster and more efficient deliveries.
Comparison of Forecasting Models
Different forecasting models offer varying levels of accuracy and complexity. Simple moving average models are easy to understand and implement but may not capture complex patterns. Exponential smoothing models give more weight to recent data, making them responsive to changes in demand. ARIMA models are more sophisticated and can handle complex patterns, but require more data and expertise to implement.
The choice of model depends on the specific needs of the business, the availability of data, and the desired level of accuracy. A company with limited historical data might opt for a simpler model like exponential smoothing, while a company with extensive data and advanced analytics capabilities might use an ARIMA model or a more complex machine learning approach.
Case Study: Preventing a Supply Chain Disruption
A major electronics manufacturer experienced recurring shortages of a critical component, leading to production delays and lost revenue. By implementing a predictive analytics system that analyzed historical supplier performance data, market trends, and geopolitical events, the company identified a potential disruption: a natural disaster in the region where the component was manufactured. The predictive model alerted the company to the impending shortage weeks in advance, allowing them to diversify their sourcing, secure alternative suppliers, and build up inventory.
This proactive approach prevented a significant disruption to their supply chain, saving millions of dollars in lost revenue and production costs. This demonstrates how predictive analytics can provide early warning systems, enabling companies to mitigate risks and ensure business continuity.
Supply Chain Risk Management with BI
Supply chain risk management is crucial for maintaining business continuity and profitability. Unforeseen events, from natural disasters to geopolitical instability, can severely disrupt operations. Business intelligence (BI) offers powerful tools to proactively identify, assess, and mitigate these risks, leading to a more resilient and efficient supply chain. By leveraging data analysis and predictive modeling, companies can transform reactive risk management into a proactive, data-driven strategy.BI’s ability to analyze vast datasets from across the supply chain provides a comprehensive view of potential vulnerabilities.
This allows for a more informed decision-making process, enabling businesses to anticipate and prepare for disruptions before they impact operations.
Potential Supply Chain Risks and BI-Driven Mitigation
A typical supply chain faces numerous risks, including supplier failures (financial distress, production disruptions), geopolitical instability (trade wars, sanctions), natural disasters (earthquakes, floods), logistical challenges (port congestion, transportation delays), and cybersecurity threats (data breaches, ransomware attacks). BI can mitigate these risks by providing real-time visibility into key performance indicators (KPIs). For example, analyzing supplier performance data can identify financially unstable suppliers at risk of default, allowing for proactive diversification of sourcing.
Similarly, monitoring geopolitical events and their potential impact on transportation routes enables businesses to adjust logistics strategies accordingly, avoiding potential delays or disruptions. Predictive analytics, powered by BI, can forecast potential disruptions based on historical data and external factors, enabling proactive mitigation strategies.
BI’s Role in Detecting and Responding to Supply Chain Disruptions, Business intelligence solutions for supply chain optimization
Business intelligence plays a vital role in both detecting and responding to supply chain disruptions. By continuously monitoring real-time data from various sources – including inventory levels, transportation schedules, and supplier performance – BI systems can identify anomalies and potential disruptions early on. For instance, an unexpected spike in lead times from a key supplier might signal an impending problem.
BI dashboards provide immediate alerts, enabling rapid response and minimizing the impact of the disruption. Furthermore, BI’s analytical capabilities allow for a rapid assessment of the disruption’s scope and impact, enabling the organization to prioritize mitigation efforts and allocate resources effectively.
Improving Supplier Relationship Management and Risk Reduction with BI
BI enhances supplier relationship management (SRM) by providing data-driven insights into supplier performance, reliability, and risk profiles. Analyzing historical data on delivery times, quality control, and communication responsiveness allows for a more objective assessment of supplier capabilities. This data-driven approach enables businesses to identify and prioritize high-performing, low-risk suppliers, fostering stronger partnerships and reducing overall supply chain risk.
BI can also identify potential areas for improvement in supplier performance, facilitating collaborative problem-solving and continuous improvement initiatives. Regular reporting and analysis through BI dashboards ensure transparency and accountability, strengthening the relationship with key suppliers.
Scenario: A Supply Chain Disruption and BI-Aided Recovery
Imagine a major earthquake disrupts a key manufacturing facility in a crucial supplier’s location. Without BI, the impact could be devastating, leading to production halts and significant financial losses. However, with a robust BI system in place, the company can quickly identify the disruption through real-time monitoring of supplier performance data and inventory levels. The BI system’s predictive analytics can then model the potential impact on the overall supply chain, identifying alternative sourcing options and suggesting contingency plans.
The organization can rapidly shift orders to backup suppliers, adjust production schedules, and proactively communicate with customers about potential delays, minimizing negative impact and maintaining customer relationships. The BI system provides data-driven insights for swift recovery, reducing downtime and minimizing financial losses.
Improving Supply Chain Visibility and Collaboration
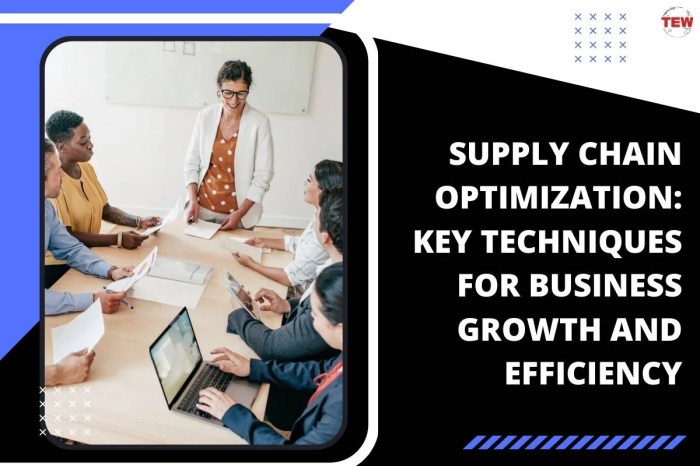
Business Intelligence (BI) solutions revolutionize supply chain management by dramatically improving transparency and fostering seamless collaboration. By centralizing and analyzing data from various sources, BI provides a holistic view of the entire supply chain, from raw material sourcing to final product delivery, empowering businesses to make data-driven decisions and optimize operations.BI enhances transparency by providing a single source of truth for all supply chain activities.
This eliminates data silos and inconsistencies, allowing all stakeholders – from manufacturers and suppliers to distributors and retailers – to access real-time information on inventory levels, order status, shipment tracking, and other critical metrics. This unified view allows for proactive identification and resolution of potential bottlenecks or disruptions before they impact the bottom line.
Real-Time Data Sharing and Collaboration Benefits
Real-time data sharing is the cornerstone of effective supply chain collaboration. The ability to instantly access and share information across the entire network significantly reduces delays and improves responsiveness. For example, if a supplier experiences a production delay, this information can be immediately communicated to downstream partners, allowing them to adjust their schedules and avoid stockouts or missed deadlines.
This proactive approach minimizes disruptions and improves overall efficiency. The benefits extend beyond simple information sharing; real-time data fuels predictive analytics, allowing businesses to anticipate potential problems and implement preventative measures.
Examples of BI-Facilitated Communication and Coordination
Consider a scenario where a retailer experiences unexpectedly high demand for a particular product. With BI-powered dashboards, the retailer can immediately see the inventory levels at the distribution center and the manufacturer’s production capacity. This information allows for immediate communication with the manufacturer to increase production or expedite shipments, ensuring customer demand is met and preventing lost sales. Similarly, if a shipment is delayed due to unforeseen circumstances (e.g., severe weather), BI can automatically alert all relevant parties, allowing them to proactively explore alternative solutions, such as rerouting the shipment or sourcing alternative suppliers.
BI System for Enhanced Manufacturer-Retailer Communication
A system leveraging BI to enhance communication between manufacturers and retailers could include a shared, cloud-based platform with real-time dashboards displaying key performance indicators (KPIs) such as inventory levels, sales forecasts, order fulfillment rates, and on-time delivery performance. This platform would integrate data from Enterprise Resource Planning (ERP) systems, Supply Chain Management (SCM) systems, and other relevant sources. Automated alerts could be triggered based on predefined thresholds (e.g., low inventory levels, production delays), ensuring timely communication and proactive problem-solving.
Furthermore, collaborative tools, such as integrated messaging and video conferencing capabilities, could facilitate direct communication and expedite decision-making. This system would promote transparency, foster trust, and optimize the entire supply chain. For example, a sudden spike in demand for a product detected by the retailer’s BI system would automatically trigger an alert to the manufacturer, enabling them to adjust production schedules and avoid potential stockouts.
The system would also track and analyze the effectiveness of these collaborative efforts, providing valuable insights for continuous improvement.
Cost Reduction and Efficiency Gains through BI
Business Intelligence (BI) isn’t just about gathering data; it’s about transforming raw information into actionable insights that drive significant cost reductions and efficiency improvements across the entire supply chain. By leveraging advanced analytics and visualization tools, businesses can pinpoint bottlenecks, optimize resource allocation, and ultimately, boost their bottom line. This section explores how BI achieves these crucial gains.BI pinpoints hidden costs and inefficiencies within the supply chain, leading to significant savings.
Through detailed analysis of historical data, current performance metrics, and predictive models, BI solutions identify areas ripe for optimization. For instance, analyzing transportation costs reveals opportunities to consolidate shipments, negotiate better rates with carriers, or optimize routing for fuel efficiency. Similarly, inventory management analysis can highlight slow-moving items, enabling better stock control and minimizing storage costs. Predictive modeling can forecast demand more accurately, reducing the risk of overstocking or stockouts, both of which are major cost drivers.
Identifying Cost Reduction Opportunities
BI tools provide a holistic view of the supply chain, allowing for the identification of specific cost reduction opportunities. This involves analyzing data from various sources, including procurement, manufacturing, logistics, and sales. For example, identifying suppliers with consistently higher prices compared to market rates allows for renegotiation or a switch to more cost-effective alternatives. Analyzing transportation data can reveal inefficient routes or modes of transport, enabling optimization and reducing fuel consumption and delivery times.
Moreover, BI can highlight areas where automation can reduce labor costs and improve accuracy.
Improved Operational Efficiency and Resource Allocation
Improved operational efficiency is a direct consequence of effective resource allocation. BI facilitates this by providing data-driven insights into resource utilization across the supply chain. For example, analyzing production data can reveal bottlenecks in the manufacturing process, allowing for adjustments to optimize throughput and reduce downtime. Similarly, analyzing warehouse data can reveal inefficiencies in storage and order fulfillment, leading to improvements in workflow and resource deployment.
By optimizing resource allocation, businesses can reduce waste, improve productivity, and ultimately increase profitability.
Real-World Examples of Cost Reduction and Efficiency Gains
Many companies have successfully implemented BI solutions to achieve significant cost savings and efficiency improvements. For example, a major retailer used BI to optimize its inventory management, reducing stockouts by 15% and overstocking by 10%, resulting in annual savings of millions of dollars. A manufacturing company used BI to analyze its supply chain and identify opportunities for process automation, reducing production costs by 8% and improving delivery times by 12%.
These are just a few examples of how BI can drive tangible results.
Cost-Benefit Analysis of Implementing a Supply Chain BI System
Implementing a supply chain BI system involves upfront investment in software, hardware, and training. However, the long-term benefits far outweigh the initial costs. A typical cost-benefit analysis might consider factors such as reduced inventory costs, optimized transportation costs, improved production efficiency, and decreased risk of supply chain disruptions. For example, a company might invest $100,000 in a BI system, but realize annual savings of $200,000 through improved efficiency and reduced costs.
This translates to a significant return on investment (ROI) and a rapid payback period. The specific ROI will vary depending on the size and complexity of the supply chain, but the potential for significant cost savings and efficiency gains is undeniable.
A well-implemented BI system can deliver a substantial ROI, often exceeding initial investment within a short timeframe.
Case Studies: Business Intelligence Solutions For Supply Chain Optimization
Real-world examples showcase the transformative power of Business Intelligence (BI) in supply chain optimization. Seeing how companies have successfully implemented these systems and reaped the rewards provides invaluable insights for businesses considering similar initiatives. Analyzing these case studies reveals common challenges and effective strategies, ultimately guiding better decision-making and successful implementation.
Supply Chain BI Implementation at a Global Retailer
This case study focuses on a large multinational retailer that implemented a comprehensive supply chain BI system to improve forecasting accuracy, optimize inventory levels, and enhance overall supply chain visibility. Prior to implementation, the retailer relied on disparate data sources and manual processes, resulting in inaccurate forecasts, excessive inventory holding costs, and frequent stockouts. The new system integrated data from various sources, including point-of-sale (POS) systems, warehouse management systems (WMS), and transportation management systems (TMS), into a centralized data warehouse.
Advanced analytics tools were then used to develop more accurate demand forecasts, optimize inventory levels using sophisticated algorithms, and identify potential supply chain disruptions. The implementation involved significant changes to existing processes and required extensive training for employees. Challenges included data cleansing and integration, resistance to change from some employees, and the need for significant upfront investment.
These challenges were overcome through a phased implementation approach, robust change management strategies, and ongoing communication and support. The success of the implementation was attributed to strong executive sponsorship, a clearly defined project scope, and a dedicated project team with the necessary expertise.
Challenges Faced and Solutions Implemented
Data integration proved to be a significant hurdle. The retailer had legacy systems with varying data formats and structures. This was addressed through a meticulous data mapping exercise and the development of ETL (Extract, Transform, Load) processes to cleanse and standardize the data before loading it into the data warehouse. Resistance to change from some employees was another challenge.
This was mitigated through comprehensive training programs, clear communication of the benefits of the new system, and ongoing support from the project team. Finally, the initial investment was substantial. However, the return on investment (ROI) was significant, with improvements in forecasting accuracy, inventory optimization, and reduced stockouts leading to substantial cost savings and increased profitability.
Key Success Factors
- Strong executive sponsorship provided the necessary resources and support.
- A clearly defined project scope ensured that the project remained focused and on track.
- A dedicated project team with the necessary expertise ensured successful implementation.
- A phased implementation approach minimized disruption to ongoing operations.
- Robust change management strategies addressed employee resistance to change.
- Ongoing communication and support ensured that employees were comfortable using the new system.
Key Takeaways
- Investing in a comprehensive supply chain BI system can lead to significant improvements in forecasting accuracy, inventory optimization, and overall supply chain visibility.
- Data integration and cleansing are critical steps in the implementation process.
- Addressing employee resistance to change is essential for successful adoption of the new system.
- Strong executive sponsorship, a clearly defined project scope, and a dedicated project team are key success factors.
- A phased implementation approach minimizes disruption to ongoing operations and allows for iterative improvements.
- The return on investment from a supply chain BI system can be substantial, leading to significant cost savings and increased profitability.