The Rise of Augmented Analytics
The future trends and predictions in the business intelligence industry – The business intelligence (BI) landscape is undergoing a dramatic transformation, driven by the increasing volume and complexity of data. Traditional BI methods, while valuable, are often hampered by their reliance on manual data preparation, complex queries, and a need for specialized expertise. Enter augmented analytics, a game-changer poised to democratize data analysis and empower a wider range of users to derive actionable insights.Augmented analytics leverages advancements in artificial intelligence (AI) and machine learning (ML) to automate many of the tedious and time-consuming tasks associated with traditional BI.
This automation allows businesses to gain faster, deeper, and more accurate insights from their data, leading to improved decision-making across all levels of the organization. The impact is far-reaching, from streamlining operational efficiency to fueling innovative product development and improving customer experiences.
Key Features of Augmented Analytics Platforms and Their Benefits
Augmented analytics platforms offer a suite of features designed to simplify and accelerate the data analysis process. These features significantly reduce the need for specialized technical skills, making advanced analytics accessible to a broader audience. Key features include automated data preparation, natural language processing (NLP) for intuitive query creation, automated insight generation, and visual data discovery tools. The benefits extend to faster time-to-insight, improved accuracy in analysis, reduced costs associated with data analysis, and increased business agility through data-driven decision-making.
For example, a retail company could use augmented analytics to automatically identify sales trends, predict future demand, and optimize inventory levels, all without requiring a dedicated team of data scientists.
Augmented Analytics vs. Traditional BI Methods
Traditional BI methods typically involve complex SQL queries, extensive data manipulation, and specialized reporting tools. This approach requires significant technical expertise and often leads to long analysis cycles. Augmented analytics, in contrast, simplifies the process through automation and user-friendly interfaces. While traditional BI excels in providing detailed, customized reports for specific needs, augmented analytics excels in quickly uncovering hidden patterns and insights from large datasets, often using visual dashboards and natural language interfaces.
Essentially, augmented analytics complements traditional BI by automating the more mundane aspects, allowing analysts to focus on higher-level strategic analysis and interpretation. Think of it as a powerful assistant to the human analyst, enhancing rather than replacing their role.
Comparison of Leading Augmented Analytics Platforms
The augmented analytics market is rapidly evolving, with several leading platforms vying for market share. The choice of platform often depends on specific business needs and existing infrastructure. Below is a comparison of three prominent players, highlighting their strengths and weaknesses:
Platform | Strengths | Weaknesses | Pricing Model |
---|---|---|---|
Tableau | Excellent visualization capabilities, user-friendly interface, strong community support. | Can be expensive for large deployments, some advanced features require significant training. | Subscription-based, tiered pricing. |
Power BI | Seamless integration with Microsoft ecosystem, cost-effective for smaller businesses, robust data connectivity. | Can be less intuitive for users unfamiliar with the Microsoft ecosystem, limited customization options compared to Tableau. | Subscription-based, tiered pricing. |
DataRobot | Powerful AI/ML capabilities, automated model building, strong focus on predictive analytics. | Steeper learning curve, requires more technical expertise than other platforms, can be expensive. | Subscription-based, enterprise-focused pricing. |
The Growing Importance of Data Storytelling: The Future Trends And Predictions In The Business Intelligence Industry
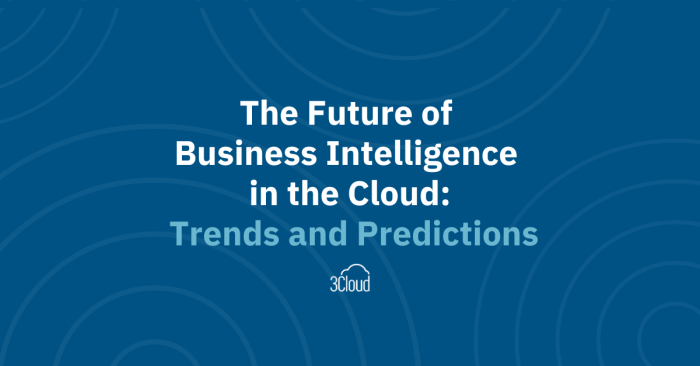
In the rapidly evolving landscape of Business Intelligence (BI), raw data is no longer enough. The ability to translate complex datasets into compelling narratives—data storytelling—is becoming a crucial skill for effective communication and driving data-driven decisions. This shift highlights the need for BI professionals to move beyond simply presenting numbers and instead focus on crafting engaging stories that resonate with their audiences, regardless of their technical expertise.Data storytelling transforms complex analytical findings into easily digestible and memorable narratives.
It bridges the gap between technical analysts and business stakeholders, ensuring everyone understands the implications of the data and can contribute to informed decision-making. By using visuals and a clear narrative structure, data storytelling makes complex information accessible, fostering a shared understanding and driving action. This approach is critical for influencing strategic direction, securing buy-in for initiatives, and ultimately, achieving business objectives.
Compelling Data Visualizations and their Role in Effective Communication
Effective data visualization is the cornerstone of compelling data storytelling. Instead of overwhelming audiences with spreadsheets or lengthy reports, strategically chosen visuals can quickly communicate key insights. For instance, a well-designed bar chart can clearly show sales performance across different regions, immediately highlighting top and bottom performers. Similarly, a line graph can effectively illustrate trends over time, such as website traffic or customer acquisition costs.
Interactive dashboards, allowing users to explore data dynamically, further enhance engagement and understanding. Consider a heatmap visualizing customer churn across different demographics; the varying color intensity instantly reveals patterns and areas needing attention. This visual representation is far more impactful than simply stating the churn rate. Another powerful visualization is a geographic map displaying sales distribution, highlighting high-potential markets or areas requiring focused marketing efforts.
These visuals provide immediate clarity and context, making complex data accessible to a broader audience.
Best Practices for Creating Engaging Data Stories
Crafting a compelling data story requires careful planning and execution. First, identify a clear narrative arc: start with a problem or question, present the data-driven insights, and conclude with actionable recommendations. Maintain a focus on the audience: tailor the language, visuals, and level of detail to their understanding and interests. Prioritize clarity and simplicity; avoid jargon and technical terms whenever possible.
Use strong visuals to support the narrative, ensuring they are accurate, relevant, and easy to interpret. Finally, always end with a clear call to action, outlining the next steps or decisions based on the insights revealed. A well-structured data story, presented with compelling visuals and a clear narrative, will not only inform but also inspire action.
Hypothetical Business Problem and Solution: A Data Story
Let’s imagine a hypothetical scenario: a retail company experiences a decline in online sales. A data story could begin by highlighting this problem with a line graph showing a downward trend in online sales over the past quarter. This immediately sets the stage. Next, a series of charts could be presented. A bar chart could break down sales by product category, revealing that sales of a particular product line are significantly lower than expected.
A geographic map could then show which regions are most affected by this decline. This geographical analysis could reveal that the drop is concentrated in a specific area, suggesting a localized issue rather than a company-wide problem. Further analysis, visualized perhaps through a pie chart, could uncover that a recent change in the company’s online marketing strategy is correlated with this drop in sales.
The solution, presented visually through a flowchart, could involve revisiting the marketing strategy and potentially adjusting it to better target the affected region. The final visualization could project an improved sales forecast based on the proposed changes. This comprehensive data story, combining various visual elements, effectively communicates the problem, its root cause, and the proposed solution in a clear and compelling manner.
The Expanding Role of AI and Machine Learning in BI
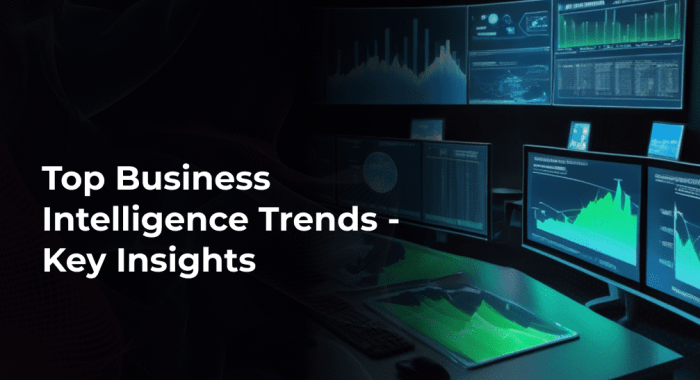
The convergence of artificial intelligence (AI) and machine learning (ML) with business intelligence (BI) is revolutionizing how businesses collect, analyze, and act upon data. No longer limited to static reports, BI is becoming a dynamic, predictive, and self-learning system, empowered by AI’s ability to uncover hidden patterns, automate complex tasks, and offer insightful predictions previously impossible to achieve. This transformation is impacting industries across the board, from finance and healthcare to retail and manufacturing.AI and machine learning are transforming BI processes in several key ways.
Examine how building a successful business intelligence team and culture can boost performance in your area.
Firstly, AI algorithms automate data preparation, cleaning, and transformation, freeing up analysts to focus on higher-level strategic tasks. Secondly, AI-powered tools can identify anomalies and outliers in data far more efficiently than human analysts, flagging potential problems or opportunities that might otherwise be missed. Thirdly, advanced machine learning models, such as neural networks and deep learning algorithms, can generate predictive insights, forecasting future trends and enabling proactive decision-making.
Finally, AI facilitates the creation of more sophisticated visualizations and dashboards, making complex data easier to understand and interpret for a wider range of users.
AI-Powered BI Tools and Their Applications
Several AI-powered BI tools are currently reshaping various industries. For example, in the financial sector, tools like those offered by companies like SAS and IBM Watson Analytics are used for fraud detection, risk management, and algorithmic trading. These tools leverage machine learning algorithms to analyze vast amounts of transactional data, identifying unusual patterns indicative of fraudulent activity or predicting market fluctuations.
In healthcare, AI-powered BI is used to analyze patient data, predict disease outbreaks, and personalize treatment plans. Companies like Google Cloud and Microsoft Azure offer platforms that integrate AI capabilities for medical image analysis and predictive modeling. In retail, AI-powered recommendation engines, similar to those used by Amazon and Netflix, personalize customer experiences and boost sales. These systems use machine learning to analyze customer behavior and predict future purchases.
The manufacturing sector benefits from predictive maintenance tools, powered by AI, that analyze sensor data from machinery to anticipate equipment failures and optimize production schedules.
Ethical Considerations in AI-Driven BI
The increasing reliance on AI in BI raises several ethical considerations. Bias in data can lead to biased outcomes, perpetuating existing inequalities. For instance, an AI-powered recruitment tool trained on historical data reflecting gender bias could inadvertently discriminate against female candidates. Data privacy and security are paramount, especially when dealing with sensitive personal information. Robust security measures and compliance with regulations like GDPR are crucial.
Transparency and explainability are also important. It’s essential to understand how AI algorithms arrive at their conclusions, to ensure accountability and prevent unintended consequences. Finally, the potential for job displacement due to automation needs careful consideration, requiring proactive strategies for workforce retraining and upskilling.
Challenges and Opportunities in AI-Driven BI
Implementing AI-driven BI solutions presents both challenges and opportunities. Challenges include the need for skilled data scientists and engineers to develop and maintain these systems, the high cost of implementation, and the potential for integration difficulties with existing BI infrastructure. Furthermore, the complexity of AI algorithms can make it difficult to understand their decision-making processes, potentially leading to a lack of trust.Opportunities, however, are abundant.
AI-driven BI can unlock new levels of insight, enabling businesses to make more informed decisions, improve operational efficiency, and gain a competitive advantage. It can automate tedious tasks, freeing up human analysts to focus on more strategic activities. AI can also personalize customer experiences, leading to increased customer satisfaction and loyalty. The potential for innovation and growth in this field is immense, promising a future where data-driven decisions are more accurate, efficient, and insightful than ever before.
The Increasing Demand for Data Literacy
In today’s data-driven world, the ability to understand, interpret, and utilize data effectively—data literacy—is no longer a luxury but a necessity. For individuals, it’s the key to navigating an increasingly complex information landscape, making informed decisions in both professional and personal life. For organizations, it’s the cornerstone of successful data-driven strategies, fostering innovation, improved decision-making, and a competitive edge.
The demand for data literacy is soaring, transforming it from a niche skill to a critical competency across all industries.Data literacy empowers individuals and organizations to harness the power of data, transforming raw numbers into actionable insights. Without it, organizations risk making decisions based on gut feeling rather than evidence, leading to missed opportunities and potentially costly mistakes.
Conversely, a data-literate workforce can identify trends, predict future outcomes, and optimize processes, driving efficiency and profitability. Consider the example of a marketing team; data literacy allows them to analyze campaign performance, understand customer behavior, and tailor strategies for maximum impact, leading to higher conversion rates and ROI.
Essential Skills and Knowledge for Data Interpretation and Analysis
Effective data interpretation and analysis requires a blend of technical and critical thinking skills. Individuals need to understand various data types (numerical, categorical, textual), be proficient in using data analysis tools (spreadsheets, BI platforms), and possess the ability to identify patterns, trends, and outliers within datasets. Furthermore, strong communication skills are crucial for conveying complex data insights to both technical and non-technical audiences.
This involves translating data into clear, concise narratives that inform decision-making. For instance, a data analyst might need to explain the implications of a complex regression analysis to a sales team, translating technical jargon into business-relevant terms.
Strategies for Improving Data Literacy Within a Business Environment
Cultivating a data-literate culture requires a multi-faceted approach. Organizations should invest in comprehensive training programs, providing employees with the necessary skills and knowledge. Furthermore, integrating data literacy into existing workflows and processes ensures that data analysis becomes a regular part of daily operations. Encouraging a culture of data exploration and experimentation, where employees feel comfortable asking questions and exploring data, is also crucial.
Finally, providing access to readily available and user-friendly data visualization tools makes data analysis more accessible and less intimidating for non-technical users. For example, a company might implement regular data literacy workshops, integrate data dashboards into their daily reporting, and encourage the use of intuitive visualization tools like Tableau or Power BI.
A Data Literacy Training Program for Employees
A comprehensive data literacy training program should encompass various learning methods to cater to diverse learning styles. The program could begin with foundational modules covering basic statistical concepts, data visualization techniques, and the use of common data analysis tools like spreadsheets. Intermediate modules could focus on more advanced analytical techniques, such as regression analysis or forecasting. Finally, advanced modules could delve into specific industry applications of data analysis, allowing employees to apply their skills to real-world business problems.Learning materials should include a mix of online courses, interactive workshops, and hands-on projects.
Suggested learning materials include online courses from platforms like Coursera or edX, interactive tutorials on data visualization tools, and case studies demonstrating real-world applications of data analysis. Assessment methods could involve quizzes, practical assignments, and presentations where employees demonstrate their ability to interpret and communicate data insights. For example, a final project could involve analyzing a real dataset relevant to the employee’s role and presenting their findings to a group.
The program’s success should be measured by employee feedback, improved decision-making processes, and ultimately, a demonstrable increase in the organization’s data-driven capabilities.
The Impact of Cloud Computing on BI
The business intelligence (BI) landscape is undergoing a dramatic transformation, largely driven by the pervasive adoption of cloud computing. This shift offers unprecedented opportunities for businesses to access powerful analytical tools and vast datasets with increased agility and scalability, but also presents unique challenges related to security and data management. Understanding the advantages and disadvantages of cloud-based BI is crucial for organizations looking to leverage data for strategic decision-making.Cloud-based BI solutions have become increasingly popular due to their inherent flexibility and cost-effectiveness.
They eliminate the need for substantial upfront investments in hardware and infrastructure, allowing businesses to focus resources on data analysis and strategic initiatives. However, reliance on third-party providers introduces concerns regarding data security and vendor lock-in, requiring careful consideration of service level agreements and data governance policies.
Advantages and Disadvantages of Cloud-Based BI Solutions, The future trends and predictions in the business intelligence industry
Cloud-based BI offers several key advantages, including reduced infrastructure costs, improved scalability and accessibility, and enhanced collaboration capabilities. Businesses can easily scale their BI resources up or down based on their needs, paying only for what they use. This pay-as-you-go model significantly reduces capital expenditure and allows for greater flexibility in managing fluctuating data demands. Furthermore, cloud-based solutions often provide better accessibility, allowing authorized users to access data and insights from anywhere with an internet connection.
However, dependence on internet connectivity, potential security vulnerabilities, and the risk of vendor lock-in are significant drawbacks. Data breaches, though rare with reputable providers, are a potential concern, and migrating data from one cloud provider to another can be complex and costly.
Comparison of Cloud Platforms for BI Applications
Several major cloud providers offer robust BI platforms, each with its own strengths and weaknesses. Amazon Web Services (AWS) offers a comprehensive suite of services, including Amazon Redshift for data warehousing and Amazon QuickSight for data visualization. Microsoft Azure provides similar capabilities with Azure Synapse Analytics and Power BI, integrating seamlessly with other Microsoft products. Google Cloud Platform (GCP) offers BigQuery, a highly scalable data warehouse, and Looker, a powerful business intelligence platform.
The choice of platform often depends on existing IT infrastructure, organizational expertise, and specific business requirements. For example, a company heavily invested in the Microsoft ecosystem might find Azure’s integration with existing tools more appealing, while a company prioritizing cost optimization might favor a more granular pricing model offered by AWS or GCP.
Security and Scalability Considerations for Cloud-Based BI Deployments
Security is paramount when deploying cloud-based BI solutions. Organizations must implement robust security measures, including data encryption, access controls, and regular security audits, to protect sensitive data. Compliance with relevant data privacy regulations, such as GDPR and CCPA, is also critical. Scalability is another key consideration. Cloud-based BI platforms should be able to handle increasing data volumes and user demands without performance degradation.
Careful planning and selection of appropriate cloud services are crucial to ensure that the BI solution can scale effectively to meet future needs. For instance, a rapidly growing e-commerce company might need a cloud platform with auto-scaling capabilities to handle peak demand during promotional periods.
Cost-Effectiveness of Cloud-Based BI Compared to On-Premise Solutions
Cloud-based BI solutions are often more cost-effective than on-premise solutions, particularly for smaller businesses or those with fluctuating data needs. The upfront costs associated with purchasing and maintaining on-premise hardware and software can be substantial. In contrast, cloud-based solutions typically involve lower upfront costs and a predictable monthly subscription fee.Consider a hypothetical scenario: a small startup needs to implement a BI system to analyze customer data.
An on-premise solution might require an initial investment of $50,000 for hardware, software licenses, and IT support, along with ongoing maintenance costs of $10,000 per year. A cloud-based solution, on the other hand, might cost $5,000 per year for a subscription service, offering significant cost savings. For larger enterprises, the cost comparison becomes more nuanced, depending on the scale of data and the complexity of the BI requirements.
However, the flexibility and scalability offered by the cloud often lead to long-term cost efficiencies even in larger organizations.
The Future of Data Visualization
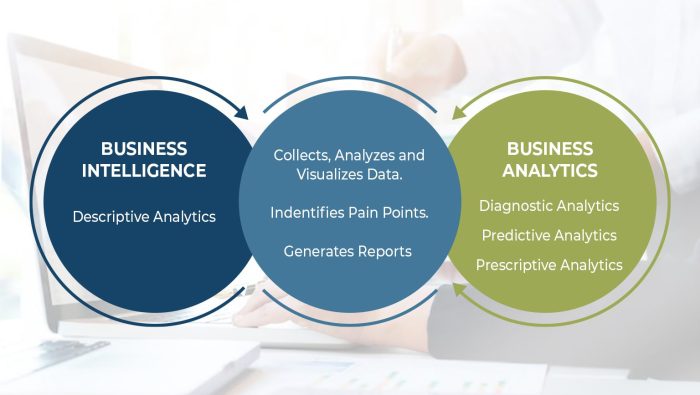
Data visualization is evolving rapidly, driven by advancements in technology and a growing need for insightful, accessible data analysis. The future of data visualization promises more interactive, immersive, and intelligent experiences, moving beyond static charts and graphs to dynamic, story-driven presentations. This evolution will significantly impact how businesses understand and utilize their data, leading to improved decision-making and strategic planning.
Emerging Trends in Data Visualization Techniques and Technologies
Several key trends are shaping the future of data visualization. These include the rise of augmented and virtual reality for immersive data exploration, the increasing sophistication of interactive dashboards, the integration of artificial intelligence for automated insight generation, and the development of more accessible and inclusive visualization tools. The use of AI in particular is poised to revolutionize the creation and interpretation of visualizations, allowing for the automatic generation of charts and graphs based on data analysis, and even the identification of unexpected patterns and trends.
This will free up data analysts to focus on higher-level tasks, such as strategic interpretation and communication of findings.
Examples of Innovative Data Visualization Methods
Interactive dashboards are already transforming how businesses monitor key performance indicators (KPIs) and track progress towards goals. These dashboards allow users to drill down into data, explore different perspectives, and customize their views to meet specific needs. For example, a marketing team might use an interactive dashboard to monitor campaign performance in real-time, adjusting strategies based on immediate feedback.
Beyond dashboards, augmented reality (AR) applications are emerging as a powerful tool for visualizing complex datasets in three-dimensional space. Imagine an AR application overlaying sales data onto a physical map of a region, allowing a sales manager to instantly identify high-performing and underperforming areas. This type of immersive visualization offers a significantly more intuitive and engaging way to understand spatial data compared to traditional methods.
Best Practices for Designing Effective and Accessible Data Visualizations
Effective data visualization is about more than just creating pretty pictures; it’s about communicating insights clearly and concisely. Best practices include prioritizing simplicity and clarity, choosing the appropriate chart type for the data, and ensuring the visualization is accessible to all users, regardless of their technical expertise or disabilities. This means using clear labels, avoiding unnecessary clutter, and employing color palettes that are both visually appealing and accessible to those with color blindness.
Furthermore, interactive elements should be intuitive and easy to use, allowing users to explore the data at their own pace and in their preferred manner. Consider the use of tooltips, clear legends, and consistent visual cues to enhance user understanding and engagement.
Projected Growth of Different Data Visualization Methods
A visual representation (described below) would show a bar graph illustrating the projected growth of different data visualization methods over the next five years. The x-axis would represent the visualization methods (e.g., interactive dashboards, augmented reality, AI-powered visualizations, traditional charts and graphs). The y-axis would represent the percentage growth. Interactive dashboards would show the highest growth, followed closely by AI-powered visualizations and AR applications.
Traditional charts and graphs would show a smaller, but still positive, growth rate, reflecting their continued relevance despite the emergence of more sophisticated techniques. This projection is based on current market trends and the anticipated adoption rates of new technologies within the business intelligence sector. For instance, the rapid increase in adoption of cloud-based BI solutions is expected to fuel the growth of interactive dashboards and AI-driven visualization tools, as these are often integrated into cloud platforms.
Similarly, the growing interest in immersive technologies will drive the adoption of AR and VR applications for data visualization, albeit at a slower pace due to the higher implementation costs and technical complexities involved.
Predictive Analytics and its Business Applications
Predictive analytics is revolutionizing how businesses operate, moving beyond simply understanding past data to anticipating future trends and outcomes. By leveraging statistical techniques and machine learning algorithms, businesses can make data-driven decisions with greater confidence and precision, ultimately leading to improved efficiency and profitability. This powerful tool allows for proactive strategies rather than reactive responses to market changes.Predictive analytics relies on historical data, statistical algorithms, and machine learning techniques to identify the likelihood of future outcomes.
It goes beyond descriptive analytics (what happened) and diagnostic analytics (why it happened) to focus on predicting whatwill* happen. This predictive capability is invaluable for informed decision-making across various business functions, from sales forecasting to risk management. The core principle lies in building models that identify patterns and relationships in existing data to forecast future events with a certain degree of probability.
Successful Implementations of Predictive Analytics Across Industries
Several industries have successfully integrated predictive analytics to enhance their operations and gain a competitive edge. For instance, in the financial sector, predictive models are used to assess credit risk, detect fraud, and personalize financial products. The healthcare industry utilizes predictive analytics for disease prediction, patient risk stratification, and optimizing resource allocation. Retail giants leverage these models for demand forecasting, inventory optimization, and personalized recommendations, significantly impacting their bottom line.
Manufacturing companies use predictive maintenance to minimize downtime and optimize production processes. Each of these examples showcases the versatility and impact of predictive analytics across diverse sectors.
Challenges in Implementing Predictive Analytics Models
Despite its immense potential, implementing predictive analytics models presents several challenges. Data quality is paramount; inaccurate or incomplete data can lead to unreliable predictions. The complexity of building and maintaining these models requires specialized skills and expertise, often necessitating investment in training and technology. Furthermore, interpreting the results and communicating them effectively to stakeholders who may not have a strong analytical background can be challenging.
Finally, ethical considerations around data privacy and bias in algorithms must be carefully addressed to ensure responsible and equitable application of predictive analytics.
A Predictive Model for Optimizing Retail Inventory Management
A conceptual predictive model for optimizing inventory management in a retail setting could utilize historical sales data, seasonality trends, promotional calendars, and external factors like weather patterns. The model would incorporate time series analysis to forecast future demand for each product. Machine learning algorithms, such as ARIMA or Prophet, could be employed to identify patterns and seasonality in sales data.
This forecast would then be integrated with lead times from suppliers and inventory levels to determine optimal order quantities. The model would also incorporate safety stock levels to account for unexpected demand fluctuations. The ultimate goal is to minimize holding costs while ensuring sufficient stock to meet customer demand and avoid stockouts. This dynamic model would continuously learn and adapt based on new data, improving its accuracy over time.
Key performance indicators (KPIs) such as inventory turnover, stockout rates, and holding costs would be monitored to assess the model’s effectiveness.