Personalized Customer Experiences
Real world applications of big data in customer relationship management – Big data is revolutionizing customer relationship management (CRM) by enabling hyper-personalization on a scale never before imagined. This level of personalization goes beyond simple segmentation; it allows businesses to understand individual customer needs and preferences with unprecedented accuracy, leading to improved customer satisfaction and increased loyalty. This is achieved by leveraging the vast amounts of data collected from various sources, analyzed to create detailed customer profiles and predict future behaviors.Big data allows for the creation of highly detailed customer profiles.
This goes beyond basic demographics; it incorporates purchase history, browsing behavior, social media interactions, and even real-time location data. This comprehensive view allows businesses to tailor their interactions with each customer to their specific needs and preferences, creating a truly personalized experience.
Tailoring Marketing Campaigns Based on Individual Preferences
By analyzing customer data, businesses can segment their audience into highly specific groups with similar preferences. For instance, a clothing retailer might identify a group of customers who frequently purchase athletic wear and show a preference for sustainable brands on social media. This allows the retailer to target this group with highly relevant marketing campaigns featuring new sustainable athletic wear, increasing the likelihood of conversion.
Discover more by delving into effective strategies for big data cost optimization and resource management further.
Similarly, a streaming service might analyze viewing history to recommend specific shows or movies, boosting engagement and reducing churn. Imagine a scenario where a coffee shop uses data to identify customers who frequently purchase lattes with oat milk and sends them a targeted promotion for a new oat milk-based seasonal beverage.
Predictive Analytics and Proactive Customer Service
Predictive analytics, a powerful application of big data, allows businesses to anticipate customer needs and proactively offer solutions. By analyzing past behavior and identifying patterns, businesses can predict potential problems or opportunities. For example, a telecommunications company might predict which customers are likely to churn based on their usage patterns and proactively offer them a retention deal. Similarly, an e-commerce company might predict which products a customer is likely to purchase next based on their browsing history and offer personalized recommendations.
This proactive approach significantly improves customer satisfaction and loyalty.
Improving Customer Service Interactions with Big Data
Imagine a customer calling a tech support hotline. Before the customer even speaks, the support agent’s screen displays a comprehensive history of the customer’s interactions with the company, including past support requests, device information, and purchase history. This allows the agent to quickly understand the customer’s situation and offer a personalized and efficient solution. The agent might even proactively suggest solutions based on predictive analytics, such as recommending a software update to prevent future issues.
This scenario highlights how big data empowers customer service agents to provide faster, more accurate, and more personalized support, dramatically improving the customer experience. The agent can address the customer by name, acknowledging their past interactions, and immediately offer relevant solutions, fostering a sense of trust and individual attention.
Enhanced Customer Segmentation
Big data analytics revolutionizes customer relationship management (CRM) by enabling businesses to move beyond basic demographic segmentation. The ability to analyze vast datasets allows for incredibly granular and insightful customer profiling, leading to more effective marketing strategies and improved customer satisfaction. This detailed segmentation goes far beyond simple age and location, offering a much clearer understanding of individual customer needs and preferences.
Several methods exist for segmenting customers using big data analytics, each with its own strengths and weaknesses. The choice of method often depends on the specific business goals and the available data. These sophisticated approaches allow for the creation of highly targeted marketing campaigns, resulting in increased conversion rates and improved ROI.
Customer Segmentation Methods
Different methods leverage various data points to create distinct customer segments. RFM analysis, for example, uses Recency, Frequency, and Monetary value to categorize customers based on their purchasing behavior. This simple yet powerful method allows businesses to quickly identify their most valuable customers. More advanced techniques like clustering algorithms (like K-means) and machine learning models analyze multiple variables simultaneously to create segments based on complex patterns and relationships.
These advanced methods uncover hidden relationships within the data, revealing customer segments that might be missed by simpler approaches. Predictive modeling can even forecast future behavior, allowing businesses to proactively tailor their strategies.
Comparing Segmentation Approaches
While RFM analysis provides a quick and easy way to segment customers based on transactional data, it might overlook other crucial factors like customer demographics, online behavior, or customer service interactions. Clustering algorithms, on the other hand, can incorporate a wider range of data points, but require more technical expertise and computational resources. Machine learning models offer the most sophisticated approach, capable of identifying complex relationships and predicting future behavior, but demand significant data volume and specialized skills.
The best approach is dependent on the available resources, data quality, and specific business objectives. A company with limited resources might find RFM analysis sufficient, while a larger enterprise with extensive data and analytical capabilities might benefit from machine learning.
Improved Targeting and Campaign Efficiency
Effective customer segmentation directly translates to improved targeting and campaign efficiency. By understanding the unique characteristics of each segment, businesses can tailor their messaging and offers to resonate with specific customer needs and preferences. For example, a company might segment its customers into “high-value,” “loyal,” and “at-risk” groups. They could then develop targeted campaigns to retain high-value customers, reward loyal customers, and re-engage at-risk customers with personalized offers.
This targeted approach minimizes wasted resources on irrelevant campaigns and maximizes the impact of marketing efforts. A campaign targeting high-value customers with exclusive offers will likely have a higher conversion rate than a generic campaign sent to the entire customer base.
Customer Segment Characteristics
Segment Name | Demographic Characteristics | Behavioral Characteristics | Value/Potential |
---|---|---|---|
High-Value Customers | High income, professional occupation | Frequent purchases, high average order value, positive reviews | High lifetime value, brand advocates |
Loyal Customers | Diverse demographics | Regular purchases, consistent engagement, positive feedback | High retention rate, positive word-of-mouth |
At-Risk Customers | Varied demographics | Decreased purchase frequency, negative reviews, long inactivity | Potential for churn, requires re-engagement |
New Customers | Varied demographics | First-time purchases, exploring products/services | High potential for growth, requires onboarding and engagement |
Improved Customer Retention
Big data analytics offers powerful tools for significantly improving customer retention rates. By leveraging the vast amounts of data collected on customer behavior, preferences, and interactions, businesses can identify at-risk customers, proactively engage them, and ultimately strengthen customer loyalty. This leads to increased profitability and sustainable business growth. Understanding and applying these data-driven strategies is crucial for success in today’s competitive market.Identifying at-risk customers is the first step towards effective retention.
Through sophisticated analysis of customer data, businesses can pinpoint those most likely to churn. This isn’t about guesswork; it’s about leveraging the power of predictive analytics.
Identifying At-Risk Customers Using Big Data Insights
Several methods exist for identifying at-risk customers using big data. These methods often combine various data points to create a comprehensive profile of customer behavior and risk. For example, a decrease in purchase frequency, a decline in engagement with marketing communications, or an increase in negative feedback can all be indicators of potential churn. Sophisticated algorithms analyze these signals, assigning risk scores to each customer, allowing businesses to prioritize their retention efforts.
A company might analyze website activity, identifying users who haven’t logged in for a significant period or haven’t interacted with new content. Similarly, analyzing customer service interactions can reveal patterns of dissatisfaction or unresolved issues that predict churn.
Proactive Engagement with At-Risk Customers
Once at-risk customers are identified, proactive engagement is key. This might involve personalized email campaigns offering exclusive discounts or promotions, tailored recommendations based on past purchases, or proactive outreach from customer service representatives to address any concerns. For instance, a subscription service might offer a free month of service to a customer whose subscription is about to expire and whose engagement has recently declined.
Or, a retailer might send a targeted email offering a discount on items similar to those a customer previously purchased but hasn’t recently bought. These targeted interventions aim to re-engage customers and prevent churn. This personalized approach is far more effective than generic marketing blasts.
Improving Customer Loyalty Programs with Big Data
Big data enhances customer loyalty programs by enabling hyper-personalization. Instead of generic rewards, programs can be tailored to individual customer preferences and behavior. For instance, a coffee shop chain could use data to offer a free pastry to customers who frequently purchase their coffee but rarely buy pastries. Data analysis can also reveal the most effective rewards and incentives, allowing businesses to optimize their loyalty programs for maximum impact.
Analyzing redemption rates, point accumulation patterns, and customer feedback allows for continuous improvement and adjustments. For example, if data shows that customers are not redeeming points for a particular reward, the program can be adjusted to offer more appealing options.
Predictive Modeling for Customer Retention
Predictive modeling is a powerful tool for retaining valuable customers. By analyzing historical data on customer behavior, demographics, and purchase patterns, businesses can build models that predict the likelihood of churn for individual customers. This allows for targeted interventions and proactive engagement strategies. For example, a telecommunications company might use predictive modeling to identify customers who are likely to switch providers based on their usage patterns, call center interactions, and online activity.
The model might then suggest targeted offers or retention strategies to prevent churn. This proactive approach is far more cost-effective than reactive measures taken after a customer has already churned.
Optimized Customer Acquisition
Big data analytics revolutionizes customer acquisition by providing unprecedented insights into customer behavior, preferences, and market trends. This allows businesses to move beyond guesswork and implement highly targeted, efficient acquisition strategies, maximizing return on investment (ROI) and minimizing wasted resources. By leveraging the power of data, companies can identify the most promising avenues for growth and cultivate lasting relationships with their ideal customers.Big data enables businesses to pinpoint the most effective channels for attracting new customers.
This involves analyzing vast datasets encompassing website traffic, social media engagement, marketing campaign performance, and customer demographics. This analysis identifies patterns and correlations that reveal which channels are most effective at converting leads into paying customers, allowing for strategic resource allocation and improved marketing campaign design.
Identifying Effective Customer Acquisition Channels
Analyzing various data sources reveals the effectiveness of different acquisition channels. For example, a company might discover that its social media marketing campaigns on platforms like Instagram generate significantly higher conversion rates compared to email marketing. This insight allows the company to reallocate budget towards more successful channels, boosting ROI and improving overall efficiency. Similarly, analyzing website analytics can identify which landing pages are most effective at converting visitors into leads, helping optimize website design and content strategy for better results.
Analyzing data from past marketing campaigns can also reveal the optimal time to run advertisements or send promotional emails. For example, data might indicate that campaigns run on Tuesdays tend to receive higher engagement than those run on weekends.
Targeted Customer Segmentation and Channel Selection
Once effective channels are identified, big data facilitates the creation of highly targeted customer acquisition plans. By segmenting customers based on demographics, purchase history, online behavior, and other relevant factors, businesses can tailor their messaging and channel selection to resonate with specific groups. For instance, a company selling luxury goods might target high-net-worth individuals through exclusive online advertising and personalized email campaigns, while simultaneously employing social media marketing to reach a broader audience.
This precision targeting increases the likelihood of converting leads into customers and reduces wasted marketing spend.
Improving Marketing Campaign Effectiveness with Big Data
Big data significantly enhances the effectiveness of marketing campaigns by enabling real-time adjustments and optimizations. By continuously monitoring campaign performance metrics such as click-through rates, conversion rates, and return on ad spend (ROAS), businesses can identify areas for improvement and make necessary changes promptly. A/B testing, powered by big data, allows for the comparison of different campaign variations to determine which approach yields the best results.
For example, a company might test different ad copy or visuals to see which version generates higher click-through rates. This iterative approach ensures that marketing campaigns are constantly refined and optimized for maximum impact.
Evaluating the ROI of Customer Acquisition Strategies
A robust process for evaluating the ROI of different customer acquisition strategies is crucial for maximizing profitability. Big data provides the tools to accurately measure the return on investment for each strategy. By tracking key metrics such as customer acquisition cost (CAC), customer lifetime value (CLTV), and the overall return on marketing investment (ROMI), businesses can gain a clear understanding of which strategies are most profitable.
For example, if a company discovers that its social media campaigns have a low CLTV despite a high CAC, it can adjust its strategy to focus on channels with higher customer lifetime value. This data-driven approach ensures that resources are allocated to the most profitable acquisition channels, driving sustainable growth.
Streamlined Customer Service
Big data analytics revolutionizes customer service, transforming it from a reactive function to a proactive, predictive powerhouse. By analyzing vast amounts of customer interaction data, businesses gain unprecedented insights into customer needs, pain points, and preferences, leading to faster resolution times, increased efficiency, and ultimately, higher customer satisfaction. This data-driven approach allows for a more personalized and efficient customer service experience.Big data significantly improves the efficiency of customer service operations by automating tasks, identifying trends, and personalizing interactions.
This leads to reduced wait times, improved first-contact resolution rates, and a more satisfied customer base. The ability to analyze historical data, predict potential issues, and proactively address them before they escalate is a game-changer for customer service departments.
Faster Issue Resolution Through Data Analysis
Analyzing past customer service interactions reveals common problems and their solutions. For example, a telecom company might discover through big data analysis that a specific phone model consistently experiences connectivity issues after a software update. Armed with this knowledge, the company can proactively contact affected customers, offer solutions, and prevent a flood of support tickets. This proactive approach minimizes customer frustration and improves overall service efficiency.
Similarly, a retailer could identify that returns for a specific product are unusually high due to a packaging flaw, leading to immediate corrective action.
Predictive Modeling for Proactive Service
Big data enables predictive modeling to anticipate potential customer service issues. By analyzing factors like past purchase history, product usage patterns, and customer demographics, businesses can identify customers at high risk of churning or experiencing technical difficulties. For example, a streaming service might predict that a customer is likely to cancel their subscription based on their viewing habits and recent customer support interactions.
This allows the company to proactively reach out to the customer, offer incentives, or address potential concerns before they lead to churn. This predictive capability is key to proactive customer retention.
Improved Customer Satisfaction Through Personalized Service
Leveraging big data to personalize customer service interactions dramatically improves satisfaction. By analyzing individual customer profiles, companies can tailor their responses to meet specific needs and preferences. Imagine a customer contacting support regarding a technical issue. A data-driven system can access the customer’s past interactions, product usage history, and even social media activity to understand their technical proficiency and communication style.
This allows the support agent to provide a more tailored and efficient solution, leading to higher customer satisfaction. This personalized approach fosters loyalty and improves the overall brand perception.
Fraud Detection and Prevention

Big data analytics has revolutionized fraud detection and prevention in customer relationship management (CRM). The sheer volume, variety, and velocity of data generated by customer interactions provide a rich landscape for identifying suspicious patterns and behaviors indicative of fraudulent activities. This allows businesses to proactively mitigate risks and protect both their bottom line and their customers’ trust.By leveraging the power of big data, businesses can move beyond reactive fraud management—simply responding to detected fraud—to a proactive, predictive model.
This shift allows for the prevention of fraud before it even occurs, saving significant resources and minimizing negative impacts on the business and its customers.
Machine Learning Algorithms in Fraud Detection, Real world applications of big data in customer relationship management
Machine learning (ML) algorithms are crucial for effectively analyzing the massive datasets involved in fraud detection. These algorithms can identify complex relationships and anomalies that would be impossible for humans to spot manually. For example, supervised learning algorithms, trained on historical data of fraudulent and legitimate transactions, can learn to classify new transactions with high accuracy. Unsupervised learning techniques, on the other hand, can identify clusters of unusual activity that might indicate emerging fraud schemes.
Commonly used algorithms include decision trees, support vector machines (SVMs), and neural networks, each offering unique strengths in identifying different types of fraud. The choice of algorithm depends on the specific type of fraud being targeted and the characteristics of the available data. For instance, neural networks are particularly effective at identifying complex, evolving patterns in transaction data, while decision trees can provide more interpretable results, facilitating easier understanding of the fraud detection process.
Hypothetical Scenario: Big Data in Fraud Detection
Imagine a large online retailer processing millions of transactions daily. Their big data system analyzes various data points, including transaction amounts, locations, times, customer purchasing history, IP addresses, and device information. A machine learning model, trained on past fraudulent transactions, flags a suspicious pattern: a new customer from an unusual location is making a series of high-value purchases using a newly issued credit card, with shipping addresses different from the billing address.
This combination of factors, identified by the algorithm as a high-risk profile, triggers an automated alert, allowing the retailer to investigate the transaction further and potentially prevent a significant loss. The system might then block the transaction pending verification, contact the customer for confirmation, or initiate a more thorough fraud investigation.
Best Practices for Using Big Data to Prevent Fraud
Effective use of big data for fraud prevention requires a strategic approach. Here are some best practices:
- Data Quality and Enrichment: Ensure data accuracy and completeness. Integrate data from multiple sources to create a comprehensive view of customer behavior.
- Real-time Analytics: Implement real-time processing of data to detect and respond to fraudulent activity immediately.
- Model Monitoring and Retraining: Regularly monitor the performance of machine learning models and retrain them with new data to maintain accuracy and adapt to evolving fraud techniques.
- Collaboration and Information Sharing: Share information and insights with other organizations to identify and combat emerging fraud trends.
- Compliance and Regulation: Adhere to all relevant regulations and best practices regarding data privacy and security.
Real-time Customer Insights
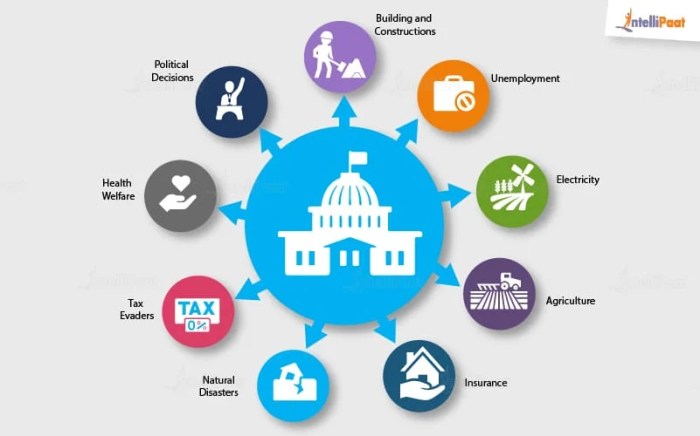
In today’s fast-paced digital world, businesses need to understand their customers in real-time to stay competitive. Real-time customer insights, powered by big data analytics, offer a significant advantage by enabling immediate responses to customer needs and proactive problem-solving. This allows for more effective decision-making and a significant improvement in customer experience.Real-time data analysis significantly improves decision-making in CRM by providing an up-to-the-minute view of customer behavior and preferences.
This eliminates the lag time associated with traditional reporting methods, allowing businesses to respond quickly to changing market trends and individual customer needs. Instead of relying on outdated data, businesses can make informed choices based on current customer interactions, resulting in more targeted campaigns, personalized offers, and improved customer service.
Real-time Data Response to Customer Needs
Using real-time data to respond to customer needs immediately is crucial for building loyalty and trust. For example, a telecommunications company might use real-time data to identify customers experiencing network issues. By detecting a sudden increase in dropped calls or slow internet speeds in a specific area, the company can proactively dispatch technicians or alert engineers to investigate and resolve the problem before customers even contact support.
Similarly, an e-commerce platform can use real-time data to identify abandoned shopping carts. They can then send personalized reminders or targeted discounts to encourage customers to complete their purchases, directly addressing a potential point of customer friction. These immediate responses demonstrate a commitment to customer satisfaction and improve conversion rates.
Benefits of Real-time Dashboards for Monitoring Customer Interactions
Real-time dashboards offer a centralized view of key customer metrics, providing a comprehensive overview of customer interactions across various channels. These dashboards can track metrics such as website traffic, social media engagement, customer support interactions, and sales conversions. This allows businesses to quickly identify trends, spot potential problems, and measure the effectiveness of marketing campaigns in real-time. For instance, a real-time dashboard could show a sudden spike in negative social media mentions, allowing the company to immediately address the issue and prevent further damage to its reputation.
The ability to monitor these interactions in real-time facilitates immediate responses and minimizes negative impacts.
Best Practices for Implementing a Real-time Data Analytics System for CRM
Implementing a real-time data analytics system requires careful planning and execution. It begins with identifying key performance indicators (KPIs) relevant to the business and its customers. Next, a robust data infrastructure capable of handling large volumes of data in real-time is essential. This involves integrating data from various sources, such as CRM systems, website analytics, social media platforms, and customer support systems.
Data quality is paramount; inaccurate or incomplete data will lead to flawed insights. Finally, a user-friendly dashboard needs to be designed to present the data in a clear and actionable manner. Regular monitoring and adjustments are crucial to ensure the system remains effective and aligned with evolving business needs. Investing in the right technology and training employees to interpret and utilize the data effectively are also key to success.
Predictive Customer Behavior Modeling: Real World Applications Of Big Data In Customer Relationship Management
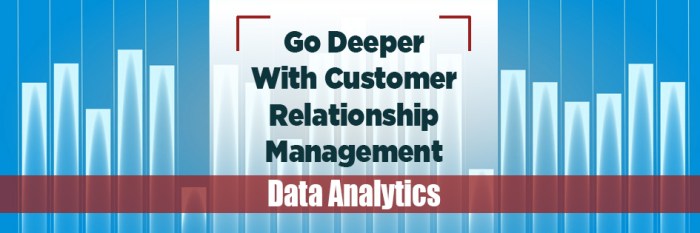
Predictive modeling leverages the power of big data to anticipate future customer actions. By analyzing vast datasets encompassing past purchases, website interactions, demographics, and more, businesses can build sophisticated models that forecast customer behavior with impressive accuracy. This allows for proactive, personalized strategies, moving beyond reactive responses to create a truly customer-centric approach.Predictive modeling uses statistical algorithms and machine learning techniques to identify patterns and trends within the data.
These patterns reveal correlations between various customer attributes and their future actions, enabling businesses to predict things like churn likelihood, product preferences, and optimal timing for marketing campaigns. The more data available, the more refined and accurate these predictions become.
Predictive Modeling for Personalized Offers and Recommendations
Predictive models are instrumental in personalizing offers and recommendations. For example, an e-commerce platform might use a model trained on past purchase history, browsing behavior, and demographic data to predict which products a customer is most likely to buy. This allows for targeted email campaigns offering discounts on those specific products, or the prominent display of those products on the customer’s homepage.
Similarly, a streaming service could predict which shows a user might enjoy based on their viewing history and preferences, leading to highly relevant content recommendations. A loyalty program might use predictive modeling to identify customers at high risk of churning and offer them personalized incentives to retain their business, such as exclusive discounts or early access to new products.
Netflix’s recommendation engine is a prime example of how this technology works in practice, offering highly personalized suggestions that drive engagement and retention.
Ethical Considerations in Using Predictive Modeling in CRM
The use of predictive modeling in CRM raises important ethical considerations. Bias in the data used to train the models can lead to discriminatory outcomes. For example, if the historical data reflects existing societal biases, the model may perpetuate and even amplify those biases in its predictions. This could lead to unfair treatment of certain customer groups. Furthermore, the transparency and explainability of these models are crucial.
Customers should have a right to understand how predictions about them are being made and to challenge those predictions if they believe they are inaccurate or unfair. Data privacy is another major concern. The collection and use of personal data for predictive modeling must comply with all relevant data protection regulations and ensure customer consent. It is crucial for businesses to implement robust data governance frameworks to mitigate these risks and ensure ethical and responsible use of predictive modeling.
Customer Journey Map Informed by Predictive Modeling
Imagine a customer journey map for a new fitness app. Instead of a generic map, this one is informed by predictive modeling. The map begins with a user downloading the app. Predictive modeling, based on the user’s signup information (age, fitness goals, etc.), forecasts their likely engagement level in the first week. If the model predicts low engagement, the map shows a proactive intervention: a personalized email with tips and encouragement, perhaps featuring a workout tailored to their stated goals.
If engagement remains low, a follow-up email with a more compelling offer (e.g., a free trial extension) is triggered. If the model predicts high engagement, the map shows a different path, suggesting in-app notifications promoting advanced features or connecting them with a virtual fitness coach. The map dynamically adjusts based on the user’s actual behavior, with the predictive model continuously refining its forecasts and adapting the journey accordingly.
The ultimate goal is personalized engagement and retention, achieved through data-driven insights and proactive interventions.