Choosing the Right Chart Type
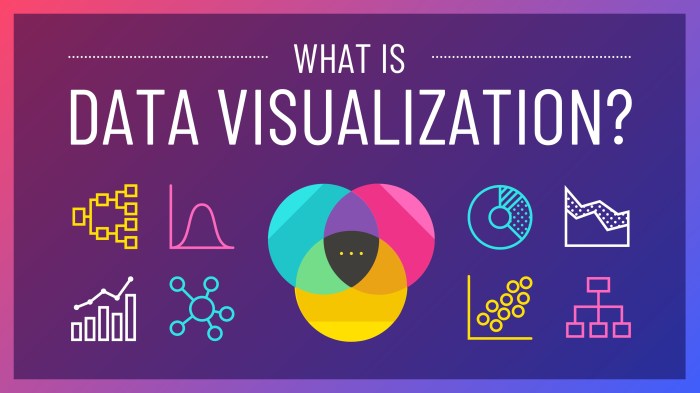
Best practices for data visualization in business intelligence – Data visualization is crucial for effective business intelligence. Choosing the right chart type is paramount to conveying insights clearly and avoiding misinterpretations. The wrong chart can obscure trends, mislead stakeholders, and ultimately hinder decision-making. Selecting the appropriate visualization depends heavily on the type of data and the message you aim to communicate.
Chart Type Selection Based on Data and Objective
Different chart types excel in showcasing specific data characteristics and answering particular business questions. A poorly chosen chart can render even the most insightful data unintelligible. Understanding the strengths and weaknesses of various chart types is essential for effective data communication.
Chart Type | Suitable Data Type | Visual Impact | Business Scenario Examples |
---|---|---|---|
Bar Chart | Categorical data, comparisons of values across categories | Clearly shows comparisons; easy to understand | Comparing sales across different regions, product performance across different quarters |
Line Chart | Time-series data, trends over time | Effectively displays trends and patterns; good for showing change over time | Tracking website traffic over a year, visualizing sales growth over multiple years |
Scatter Plot | Two numerical variables, correlation between variables | Reveals relationships and correlations; identifies outliers | Analyzing the relationship between advertising spend and sales revenue, exploring the correlation between customer age and purchase frequency |
Pie Chart | Proportional data, showing parts of a whole | Visually appealing for showing proportions; can be difficult to compare segments precisely | Illustrating market share of different brands, showing the proportion of revenue from different product categories (Use cautiously; avoid too many segments) |
Sales Trend Visualization: A Case Study
To effectively show sales trends over time for different product lines, a line chart is the most suitable choice. This chart type excels at illustrating changes in data over time, making it ideal for visualizing sales performance. Each product line would be represented by a separate line, allowing for easy comparison of their respective sales trajectories. The x-axis would represent time (e.g., months or quarters), and the y-axis would represent sales revenue.
This clear, concise visualization allows stakeholders to quickly identify top-performing products, periods of growth or decline, and potential areas for improvement. Using different colors for each product line further enhances readability and comparability. For instance, a vibrant blue line could represent Product A, a contrasting orange line for Product B, and so on. This ensures visual clarity and allows for easy identification and comparison of trends.
Data Preparation and Cleaning
Data visualization is only as good as the data it’s based on. Garbage in, garbage out, as the saying goes. Before you even think about choosing the perfect chart, you need to ensure your data is clean, accurate, and ready for prime time. This crucial step involves several key processes that will significantly impact the insights you glean from your visualizations.
Ignoring this step can lead to misleading conclusions and flawed business decisions.Data preparation and cleaning is a multi-step process that involves transforming raw data into a format suitable for visualization. This includes cleaning the data to remove errors and inconsistencies, transforming the data to make it more suitable for analysis, and aggregating the data to summarize key information. Think of it as meticulously prepping your ingredients before you start cooking – you wouldn’t bake a cake with spoiled eggs, would you?
Data Cleaning Techniques
Data cleaning involves identifying and correcting or removing errors, inconsistencies, and inaccuracies in a dataset. This might include handling missing values, dealing with outliers, and ensuring data consistency. For example, imagine a sales dataset where some entries list “USD” as the currency while others use “$”. This inconsistency needs to be rectified before visualization. A common approach is to standardize all currency notations to a single format, like “USD”.
Another example is correcting typos in categorical data, such as “New York” versus “New Yrok”.
Handling Missing Values
Missing values are a common problem in datasets. They can significantly impact the accuracy of your analysis and visualizations if not handled properly. Ignoring them can lead to biased results and misinterpretations. There are several ways to address missing data. Simple imputation methods, like replacing missing values with the mean or median of the column, are quick but can distort the distribution if a large portion of data is missing.
More sophisticated techniques include using k-Nearest Neighbors (KNN) imputation, which considers the values of similar data points to estimate the missing value. Alternatively, you might choose to remove rows or columns with excessive missing values, especially if the missing data is non-random. The best approach depends on the nature and extent of the missing data and the characteristics of your dataset.
Outlier Detection and Treatment
Outliers are data points that significantly deviate from the rest of the data. They can be caused by errors in data entry, measurement errors, or simply represent truly unusual events. Outliers can skew your visualizations and distort your analysis. Identifying outliers often involves visual inspection using box plots or scatter plots. Statistical methods, such as calculating Z-scores, can also help pinpoint outliers.
Once identified, outliers can be removed, transformed (e.g., using logarithmic transformation), or winsorized (capping extreme values at a certain percentile). The appropriate treatment depends on the context and the cause of the outlier. For instance, a single sale of $1 million in a dataset of small-scale transactions might be a legitimate outlier, whereas a data entry error resulting in a negative sales figure is clearly an error that should be corrected.
Best Practices for Data Accuracy and Consistency, Best practices for data visualization in business intelligence
Ensuring data accuracy and consistency is paramount for reliable visualizations. Here’s how:
- Establish clear data definitions and standards. This means defining exactly what each data point represents and ensuring consistent units of measurement.
- Implement data validation rules. These rules should automatically check for data inconsistencies and errors during data entry or import.
- Regularly audit your data. This involves periodically reviewing your data for errors, inconsistencies, and outliers.
- Document your data cleaning and transformation processes. This ensures reproducibility and transparency.
- Use version control for your data. This allows you to track changes to your data and revert to previous versions if necessary.
By following these best practices, you’ll significantly improve the quality of your data and, ultimately, the effectiveness of your visualizations. Remember, clean data is the foundation of impactful business intelligence.
Effective Use of Color and Visual Elements
Data visualization in business intelligence isn’t just about presenting numbers; it’s about crafting a compelling narrative that drives understanding and action. Choosing the right colors and visual elements is crucial in achieving this goal. A well-designed visualization, leveraging color and visual aids effectively, can significantly improve data interpretation and ultimately, decision-making. Conversely, poor choices can lead to misinterpretations and confusion.Color psychology plays a significant role in how we perceive data.
Certain colors evoke specific emotions and associations. For instance, red often represents urgency or negative trends, while green signifies growth or positive performance. Understanding this allows you to strategically guide the viewer’s eye and highlight key insights. Using a consistent and thoughtfully chosen color palette is essential for maintaining visual harmony and preventing the chart from becoming overwhelming or distracting.
Color Palette Selection and its Impact on Data Interpretation
Effective color palettes are crucial for clear data communication. Consider using color-blind-friendly palettes to ensure accessibility for all viewers. For example, a good choice would be a palette utilizing shades of blue, green, and orange, offering sufficient contrast while remaining easily distinguishable even for individuals with color vision deficiencies. Avoid using too many colors, as this can lead to visual clutter and make it difficult to discern patterns.
An ineffective palette might be one that uses similar shades of red and pink, making it challenging to distinguish between different data points. Remember that the goal is clarity, not artistic expression.
Utilizing Visual Elements for Enhanced Clarity
Visual elements such as labels, legends, and annotations are essential for adding context and meaning to your visualizations. These elements act as guides, helping viewers quickly grasp the information presented. Let’s illustrate this with a hypothetical bar chart showing sales figures for four different product lines (A, B, C, and D) over the past year. Each bar represents a product line’s monthly sales.
The chart’s title clearly states “Monthly Sales Performance by Product Line (2024)”. A clear legend distinguishes each product line by its assigned color (e.g., Product A: Blue, Product B: Green, Product C: Orange, Product D: Purple). Labels on each bar indicate the specific sales figures for each month and product. Finally, annotations might be used to highlight particularly strong or weak months for specific products, for example, “Record Sales for Product A in December” could be written next to the relevant bar.
Each element plays a vital role in ensuring that the data is easily understood and the key insights are readily apparent.
Guidelines for Creating Visually Appealing Charts
Creating visually appealing and easily understandable charts requires careful consideration of several factors. Here are some key guidelines:
- Prioritize clarity over aesthetics. The primary goal is to effectively communicate data, not to create a visually stunning masterpiece.
- Use a consistent color scheme throughout your visualization. Avoid using too many colors or colors that clash.
- Keep your charts simple and uncluttered. Avoid unnecessary visual elements that might distract from the data.
- Use clear and concise labels and titles. Ensure that all labels are easy to read and understand.
- Choose an appropriate chart type for your data. Different chart types are suitable for different types of data and insights.
- Ensure accessibility for all users. Use color-blind-friendly palettes and sufficient contrast between elements.
- Always test your visualizations with a diverse group of users to ensure that they are easily understood by everyone.
Accessibility and Inclusivity
Data visualization in business intelligence isn’t just about creating pretty charts; it’s about ensuring everyone can understand and benefit from the insights presented. Accessibility and inclusivity are paramount, making your visualizations usable and meaningful for a diverse audience, including those with disabilities. Ignoring this crucial aspect severely limits the impact of your hard work.Creating accessible visualizations is about more than just following guidelines; it’s about fostering a truly inclusive environment where everyone can participate and contribute.
By prioritizing accessibility, you ensure that data-driven decisions are informed by the broadest possible range of perspectives, leading to more equitable and effective outcomes. This means considering the needs of individuals with visual, auditory, motor, and cognitive impairments, as well as those from diverse cultural backgrounds.
Alternative Text and Descriptive Labels for Screen Readers
Screen readers rely heavily on alternative text (alt text) to convey the meaning of visual elements to visually impaired users. For charts, alt text should concisely describe the chart type, key data points, and overall message. For example, a bar chart comparing sales figures across different regions might have alt text like: “Bar chart showing sales performance by region.
Region A had the highest sales at $1 million, followed by Region B at $800,000, and Region C at $500,000.” Similarly, descriptive labels should be used for all chart elements, including axes, legends, and data points. These labels provide context and meaning, even for users who aren’t relying on screen readers. Avoid relying solely on color to convey information; always provide textual descriptions alongside color-coded elements.
For instance, instead of saying “The red bars represent sales in the North,” use “The bars representing sales in the North are colored red.”
Designing Culturally Sensitive and Data-Literate Visualizations
Effective data visualization transcends cultural boundaries. Consider using universally understood symbols and icons instead of culturally specific imagery. Avoid using colors that may have different meanings in various cultures. For instance, red signifies good luck in some cultures and bad luck in others. Opt for neutral color palettes and clear, concise labels.
Furthermore, tailor the complexity of your visualizations to the data literacy level of your audience. Avoid overly technical jargon or complex chart types for audiences with limited data literacy. Instead, opt for simpler visualizations like bar charts or pie charts, supplemented by clear and concise explanations. For example, if presenting to a non-technical audience, a simple bar chart illustrating key trends might be more effective than a complex scatter plot with multiple variables.
Remember, the goal is to communicate insights clearly and effectively, not to impress with technical prowess.
Storytelling with Data: Best Practices For Data Visualization In Business Intelligence
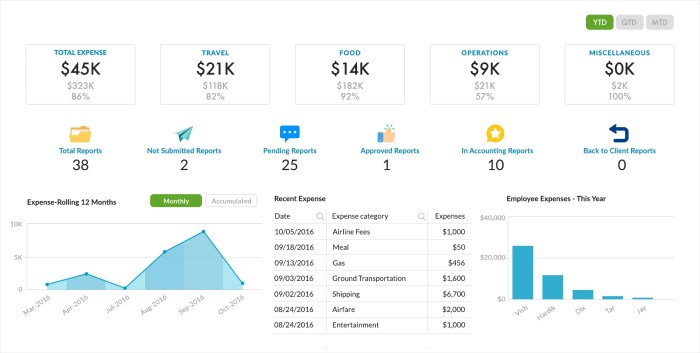
Data visualization isn’t just about presenting numbers; it’s about crafting a narrative that resonates with your audience. A compelling data story uses visuals to highlight key trends, insights, and ultimately, drive action. Think of it as translating complex data into a clear, concise, and engaging message. Effective storytelling with data transforms raw information into actionable knowledge.Data visualization allows you to communicate complex information simply and effectively, making it accessible to a broader audience.
By using visual cues to emphasize key trends and insights, you can guide your audience’s understanding and create a memorable experience. This approach moves beyond simply displaying data; it transforms data into a persuasive argument or a captivating journey of discovery.
Visualizing a Hypothetical Sales Story
Let’s imagine a hypothetical dataset tracking monthly sales of a new product over a year. A simple line chart could immediately showcase overall sales growth. A bar chart could compare sales across different regions, revealing which areas performed best and highlighting opportunities for targeted marketing. A further breakdown using a combination chart, incorporating a line graph showing sales trend alongside a bar chart comparing sales by region, provides a richer understanding of sales performance over time and by location.
This multi-faceted approach allows the audience to grasp both the overall trend and the regional nuances simultaneously.
Comparing Storytelling Approaches
Different approaches to storytelling with data visualizations exist. One common approach is the chronological approach, where data is presented in a time-series format to illustrate a trend over time. Another approach might focus on comparison, using bar charts or other comparative visualizations to highlight differences between groups or categories. A third approach could be geographic, utilizing maps to show spatial distribution of data.
The best approach depends on the data and the story you want to tell. For instance, presenting year-over-year sales growth might benefit from a line chart, while comparing market share across competitors would be better suited to a bar chart. The key is choosing the visualization that best supports the narrative and highlights the key takeaway.
Interactive Visualizations and Dashboards
Interactive dashboards are revolutionizing business intelligence, moving beyond static reports to deliver dynamic, engaging insights. They empower users to explore data independently, uncovering hidden trends and making faster, more informed decisions. This shift from passive consumption to active exploration is crucial for leveraging the full potential of data-driven insights.Interactive dashboards offer several key advantages. They allow for real-time data updates, providing a continuously refreshed view of key performance indicators (KPIs).
This dynamic nature ensures that decision-makers are always working with the most current information. Furthermore, the ability to drill down into data, filter results, and customize views allows users to personalize their analysis, focusing on the specific aspects most relevant to their needs. This personalized approach increases user engagement and ensures that data insights are easily accessible and understood.
Interactive Dashboard Elements
Several interactive elements significantly enhance user engagement and exploration within dashboards. These elements transform data visualization from a passive activity to an interactive exploration.
- Interactive Filters: Allow users to select specific data subsets based on various criteria (e.g., date range, product category, geographic location). This feature empowers users to focus their analysis and identify patterns within specific segments of the data.
- Drill-Down Functionality: Enables users to progressively explore data at increasing levels of detail. For instance, starting with an overview of total sales, a user could drill down to see sales by region, then by individual store, and finally by individual product.
- Data Selection and Highlighting: Users can select specific data points on charts or maps to view detailed information or related data. Highlighting can draw attention to outliers or key trends.
- Customizable Views: Dashboards should allow users to personalize their view by selecting which KPIs to display, rearranging elements, and adjusting chart types or scales. This ensures that the dashboard adapts to individual user preferences and analytical needs.
- Interactive Maps: Geo-spatial data visualization is enhanced with interactive maps, allowing users to zoom in on specific regions, explore geographic patterns, and pinpoint high-performing or underperforming areas.
KPI Tracking Dashboard Example
Imagine a simple interactive dashboard designed for tracking key performance indicators (KPIs) for an e-commerce business.This dashboard would feature a central area displaying key metrics like total revenue, conversion rate, average order value, and customer acquisition cost, all displayed using clear and concise charts (e.g., line charts for trends, bar charts for comparisons). To the left, a filter panel would allow users to select a date range, product category, or marketing campaign.
Selecting a specific date range would automatically update all the charts to reflect the chosen period. Clicking on a specific data point in a chart, such as a particularly high or low conversion rate on a specific day, could trigger a pop-up window providing detailed information on that day’s sales, marketing spend, and customer demographics. Finally, an interactive map would show sales distribution across different geographic regions, allowing users to identify high-performing and underperforming areas.
The entire dashboard would be designed with a clean, intuitive layout, ensuring ease of navigation and data interpretation. This interactive nature allows for a much deeper understanding of the business’s performance compared to a static report.
Maintaining Visual Consistency and Branding
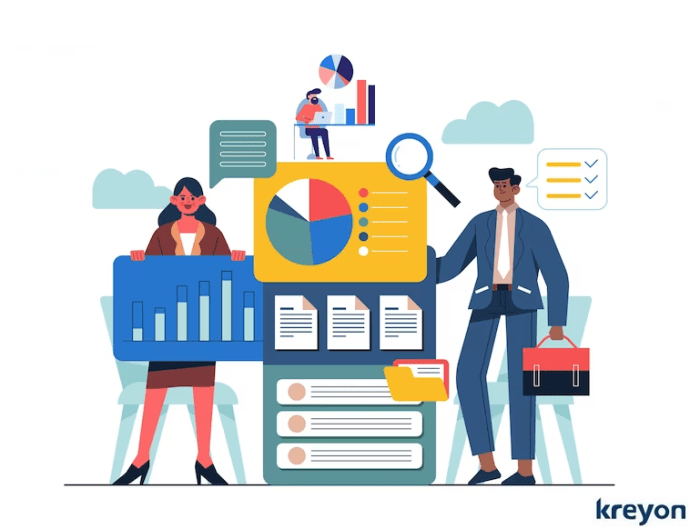
Maintaining a consistent visual style across all your business intelligence data visualizations is crucial for building a strong brand identity and ensuring clear, effective communication. A unified look and feel improves data comprehension, strengthens brand recognition, and ultimately enhances the overall impact of your data storytelling. Inconsistency, on the other hand, can lead to confusion and dilute the message you’re trying to convey.Integrating your data visualizations with existing branding guidelines ensures that your data presentations align seamlessly with your company’s overall image.
This cohesive approach strengthens brand recognition and reinforces the professional image of your organization. By adhering to established brand guidelines, you create a professional and trustworthy impression on stakeholders, regardless of the specific data being presented.
Data Visualization Style Guide for “InnovateTech”
InnovateTech, a hypothetical tech company, can implement a data visualization style guide to ensure consistency across all its data presentations. This guide will dictate the visual elements used in all charts, graphs, and dashboards.
Color Palette: InnovateTech’s primary brand colors are a deep blue (#0056b3), a vibrant teal (#00a69c), and a light grey (#f2f2f2). These colors will be used consistently across all visualizations. The deep blue will be used for primary data points, the teal for secondary data, and the light grey for backgrounds and neutral elements. A limited, carefully chosen color palette prevents visual clutter and ensures accessibility for color-blind individuals.
For example, a bar chart showing sales figures might use deep blue for the current year’s data and teal for the previous year’s data.
Font Selection: InnovateTech will utilize Open Sans as its primary font for all text elements within data visualizations. This sans-serif font is highly legible and easily readable across various screen sizes and resolutions. For headings and titles, a bolder version of Open Sans, such as Open Sans SemiBold, will be used to create visual hierarchy. The consistent use of a single font family improves readability and enhances the overall aesthetic appeal.
Chart Types: InnovateTech will prioritize chart types best suited for the data being presented. For example, line charts will be used to show trends over time, bar charts for comparisons between categories, and pie charts for showing proportions of a whole. Complex visualizations will be avoided unless absolutely necessary, prioritizing clarity and ease of understanding. A consistent approach to chart selection helps maintain visual uniformity and enhances the clarity of the data being presented.
For instance, a dashboard displaying quarterly revenue would likely employ a line chart to showcase revenue trends over time.
Logo and Branding Elements: The InnovateTech logo will be subtly incorporated into dashboards and presentations, typically in the header or footer. This reinforces brand identity without distracting from the data itself. The logo should always maintain its correct size and proportions as specified in the overall brand guidelines.