Enhanced Fraud Detection and Prevention
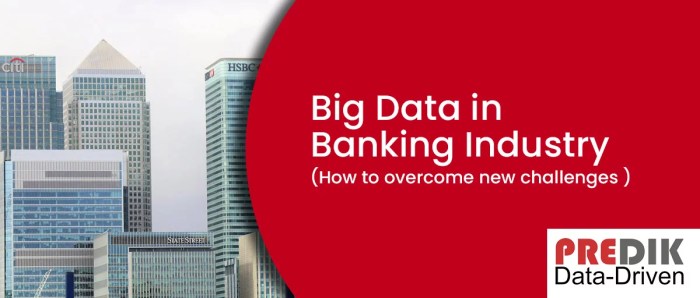
How big data is transforming the financial services industry and risk management – Big data analytics is revolutionizing fraud detection in the financial services industry, moving beyond traditional rule-based systems to a more proactive and sophisticated approach. The sheer volume, velocity, and variety of data now available allows for the identification of subtle patterns and anomalies indicative of fraudulent activity that would previously have gone unnoticed. This enhanced detection leads to significant cost savings and improved customer trust.
Big data analytics leverages advanced algorithms to sift through massive datasets, uncovering hidden connections and predicting future fraudulent behavior. This allows financial institutions to not only detect fraud more effectively but also to prevent it before it occurs. The implementation of real-time fraud detection systems using big data technologies enables immediate responses to suspicious activities, minimizing financial losses and reputational damage.
Algorithms for Identifying Fraudulent Transactions
Several sophisticated algorithms are employed to identify fraudulent transactions. Machine learning techniques, such as anomaly detection, are particularly effective. Anomaly detection algorithms identify transactions that deviate significantly from established patterns. For example, a sudden surge in transaction volume from an unusual geographic location might trigger an alert. Another powerful technique is network analysis, which examines the relationships between different accounts and transactions to detect suspicious clusters or patterns.
This approach can identify fraudulent rings or coordinated attacks. Furthermore, deep learning models, which are capable of processing complex and unstructured data, are increasingly being used to improve the accuracy and efficiency of fraud detection. These models can learn from vast datasets of past fraudulent and legitimate transactions, constantly adapting to new and evolving fraud techniques.
Real-Time Fraud Detection Systems
Real-time fraud detection systems utilize big data technologies like Hadoop and Spark to process massive volumes of transaction data in real-time. These systems incorporate sophisticated algorithms and machine learning models to analyze data streams, identify suspicious patterns, and generate alerts instantaneously. For example, a system might flag a transaction if it involves a large sum of money, an unusual location, or a device associated with previous fraudulent activity.
The speed and efficiency of these systems are crucial in minimizing financial losses and preventing further fraudulent activities. The implementation typically involves integrating various data sources, including transaction data, customer information, and external threat intelligence feeds.
Comparison of Traditional and Big Data-Driven Fraud Detection
Traditional fraud detection methods primarily rely on rule-based systems that define specific criteria for flagging suspicious transactions. While effective for detecting known fraud patterns, these systems are often inflexible and struggle to adapt to new fraud techniques. Big data-driven approaches, on the other hand, leverage advanced analytics and machine learning to identify previously unknown patterns and adapt dynamically to evolving fraud schemes.
This leads to higher detection rates and a more proactive approach to fraud prevention.
Method | Advantages | Disadvantages | Implementation Cost |
---|---|---|---|
Traditional Rule-Based Systems | Simple to implement, easy to understand | Limited adaptability, high false positive rate, struggles with new fraud types | Low |
Big Data-Driven Approaches | High detection rates, adaptability to new fraud types, proactive fraud prevention | High complexity, requires specialized expertise, high initial investment | High |
Improved Risk Assessment and Management
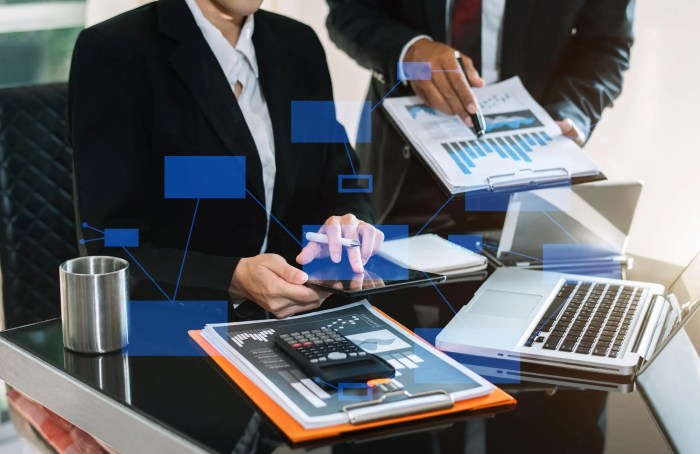
Big data analytics is revolutionizing risk assessment and management within the financial services industry, moving beyond traditional methods to offer a more comprehensive and accurate understanding of risk profiles. This enhanced understanding allows for more informed decision-making, leading to better risk mitigation strategies and ultimately, increased profitability and stability.Big data’s impact on credit risk assessment is particularly significant. By leveraging vast datasets encompassing transactional history, social media activity, and alternative data sources, financial institutions can develop far more nuanced and predictive models than ever before.
This shift from solely relying on traditional credit scores to incorporating a broader range of data points provides a more holistic view of a borrower’s creditworthiness.
Credit Risk Assessment and Mitigation Using Big Data
Big data significantly enhances credit risk assessment by enabling the analysis of a much wider array of data points than traditional methods. This includes not only historical credit data but also alternative data sources such as social media activity, online behavior, and transactional data from various platforms. For example, analyzing social media posts can reveal insights into a borrower’s financial habits and lifestyle, potentially indicating a higher or lower risk profile.
Similarly, analyzing transactional data can provide a real-time view of spending patterns and cash flow, offering a more up-to-date picture of a borrower’s financial situation. This comprehensive data analysis allows for a more accurate prediction of loan defaults and a more effective mitigation of credit risk.
Predicting Loan Defaults with Big Data
Machine learning algorithms, fueled by big data, are proving exceptionally effective in predicting loan defaults. These algorithms can identify complex patterns and relationships within the data that would be impossible for humans to detect manually. For example, a model might identify a specific combination of factors – such as a recent change in employment, increased spending on luxury goods, and a decrease in savings – as highly predictive of loan default.
This allows lenders to proactively manage risk by adjusting interest rates, loan amounts, or even denying loans altogether to borrowers identified as high-risk. One real-world example is the use of machine learning by major banks to flag potentially fraudulent applications or identify borrowers at a higher risk of default based on their digital footprint and transaction history. These predictive models have demonstrably reduced default rates and improved overall portfolio performance.
Machine Learning Models for Credit Scoring
Traditional credit scoring models often rely on limited data points, potentially overlooking crucial indicators of creditworthiness. Big data and machine learning offer a solution by enabling the development of more sophisticated credit scoring models that incorporate a far broader range of data. These models can analyze millions of data points to identify subtle patterns and correlations, resulting in more accurate and nuanced credit risk assessments.
For example, a machine learning model might incorporate data from alternative sources, such as mobile phone usage patterns or utility payment history, to create a more comprehensive credit score. This allows lenders to assess the creditworthiness of individuals who may be underserved by traditional credit scoring systems, leading to greater financial inclusion and more informed lending decisions.
Big Data Integration in Loan Approval Workflow
The integration of big data into the loan approval process can be illustrated through a streamlined workflow:
1. Data Collection and Integration
Gathering data from various sources, including credit bureaus, internal databases, and alternative data providers.
2. Data Cleaning and Preprocessing
Understand how the union of the future trends and predictions in the business intelligence industry can improve efficiency and productivity.
Transforming raw data into a usable format for analysis, handling missing values, and ensuring data quality.
3. Feature Engineering
Creating new variables from existing data to improve model accuracy, for example, combining different data points to create a comprehensive risk score.
4. Model Training and Validation
Developing and testing machine learning models using historical loan data to predict default probabilities.
5. Risk Assessment and Scoring
Applying the trained model to new loan applications to generate individual risk scores.
6. Decision-Making and Loan Approval
Using the risk scores to inform lending decisions, adjusting interest rates, loan amounts, or approving/rejecting applications based on risk levels.
7. Monitoring and Model Refinement
Continuously monitoring model performance and retraining the model with new data to maintain accuracy and adapt to changing market conditions.
Key Metrics for Risk Assessment in Lending
Understanding the key metrics used in risk assessment is crucial for effective lending. These metrics provide quantitative measures of risk and help lenders make informed decisions.
- Default Rate: The percentage of loans that end up in default.
- Probability of Default (PD): The likelihood that a borrower will default on a loan.
- Expected Loss (EL): The anticipated loss from a loan default, calculated as PD multiplied by Exposure at Default (EAD) and Loss Given Default (LGD).
- Exposure at Default (EAD): The outstanding loan balance at the time of default.
- Loss Given Default (LGD): The percentage of the loan balance that is lost in case of default.
- Return on Equity (ROE): A measure of profitability reflecting the efficiency of the lender’s use of capital.
- Net Interest Margin (NIM): The difference between interest earned on loans and interest paid on deposits.
These metrics, when analyzed in conjunction with big data insights, allow for a more precise and proactive approach to risk management in lending.
Personalized Financial Products and Services
Big data is revolutionizing the financial services industry, moving beyond generic offerings to create highly personalized experiences tailored to individual needs and preferences. This shift allows financial institutions to offer products and services that are not only more relevant but also significantly improve customer satisfaction and loyalty. The ability to analyze vast datasets allows for a granular understanding of customer behavior, financial goals, and risk tolerance, leading to a new era of customized financial solutions.The power of big data lies in its capacity to analyze diverse data points, uncovering previously hidden patterns and insights that inform the development of personalized financial products.
This includes leveraging transactional data, demographic information, social media activity, and even geolocation data to build a comprehensive profile of each customer. This detailed profile then guides the creation of bespoke financial solutions, from investment strategies to insurance plans, maximizing relevance and effectiveness.
Personalized Investment Strategies Driven by Big Data Analysis
Big data analytics enables the creation of highly personalized investment strategies by analyzing a client’s risk tolerance, investment goals, and financial history. For instance, a young professional with a high-risk tolerance and long-term investment horizon might be recommended a portfolio heavily weighted towards growth stocks, while a retiree nearing retirement might receive a more conservative allocation focusing on income-generating assets.
Algorithms can dynamically adjust portfolios based on market fluctuations and individual circumstances, ensuring optimal performance and alignment with evolving financial goals. This approach contrasts sharply with traditional, one-size-fits-all investment strategies which often fail to account for individual nuances.
Customer Segmentation Based on Big Data Insights, How big data is transforming the financial services industry and risk management
Big data allows financial institutions to segment their customer base with unprecedented accuracy. Instead of relying on broad demographic categories, institutions can now create highly targeted segments based on detailed behavioral and financial data. For example, customers might be segmented based on spending habits, investment preferences, debt levels, or even their social media engagement. This precise segmentation enables the development of highly targeted marketing campaigns and the delivery of relevant product offerings, increasing the effectiveness of sales and marketing efforts.
The result is a more efficient and profitable approach to customer acquisition and retention.
Effectiveness of Personalized Financial Advice Compared to Traditional Approaches
Personalized financial advice, powered by big data, significantly surpasses traditional approaches in terms of effectiveness and client satisfaction. Traditional methods often rely on generic advice and standardized products, failing to address the unique circumstances of individual clients. In contrast, big data-driven personalized advice offers tailored recommendations, leading to improved investment outcomes, reduced risk, and increased client engagement. Studies have shown that personalized investment strategies outperform traditional approaches, leading to higher returns and lower volatility for clients.
The increased relevance and individual attention translate to higher client satisfaction and stronger client-advisor relationships.
Big Data Enhancement of Customer Experience in Financial Services
Big data dramatically enhances customer experience in several ways. Firstly, it allows for the proactive identification and resolution of potential issues. For example, anomaly detection algorithms can flag suspicious transactions, preventing fraud and protecting customers. Secondly, personalized recommendations and offers, based on individual needs and preferences, create a more relevant and engaging experience. Finally, improved customer service through AI-powered chatbots and personalized support channels ensures efficient and timely responses to customer inquiries.
This combination of proactive risk management, tailored offerings, and responsive customer service contributes to a significantly improved overall customer experience.
Regulatory Compliance and Reporting
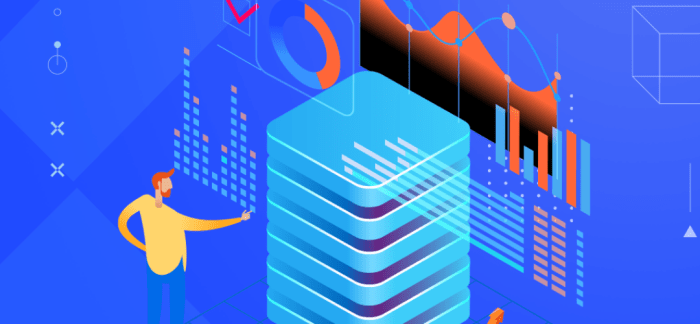
The financial services industry operates within a complex web of regulations designed to protect consumers and maintain market stability. Meeting these requirements is crucial, but the sheer volume and complexity of regulations pose significant challenges for financial institutions. Big data analytics offers a powerful solution, enabling institutions to navigate this landscape more efficiently and effectively.Big data facilitates regulatory compliance by providing the tools to analyze vast datasets, identify patterns indicative of non-compliance, and generate the necessary reports with accuracy and speed.
This ultimately reduces operational risks and enhances the institution’s reputation.
Challenges in Meeting Regulatory Requirements
Financial institutions face numerous hurdles in complying with regulations. These include the sheer volume of regulations, the constantly evolving regulatory landscape, the difficulty in integrating data from disparate sources, the need for real-time monitoring, and the potential for human error in manual processes. The penalties for non-compliance can be severe, ranging from hefty fines to reputational damage and even legal action.
The cost of compliance itself is also a significant factor, requiring substantial investment in technology and personnel.
Big Data and Anti-Money Laundering (AML) Regulations
Big data analytics plays a crucial role in enhancing AML compliance. By analyzing transactional data, customer profiles, and other relevant information, institutions can identify suspicious activities indicative of money laundering. Machine learning algorithms can detect anomalies and patterns that might escape human detection, flagging potentially suspicious transactions for further investigation. This proactive approach significantly improves the effectiveness of AML programs, reducing the risk of financial crime.
For example, analyzing transaction networks can reveal hidden connections between seemingly unrelated accounts, highlighting potential money laundering schemes.
Big Data and Know Your Customer (KYC) Compliance
KYC compliance requires financial institutions to verify the identity of their customers and understand their business activities. Big data facilitates this process by enabling automated identity verification using various data sources, including government databases, credit bureaus, and social media. This automated approach accelerates the onboarding process and reduces manual effort. Moreover, big data allows for continuous monitoring of customer activity, enabling institutions to detect any changes in customer behavior that may indicate increased risk.
For instance, a sudden increase in high-value transactions from a low-risk customer might trigger an alert, prompting further investigation.
Big Data and Regulatory Reporting
Generating accurate and timely regulatory reports is a critical aspect of compliance. Big data analytics streamlines this process by automating data aggregation and analysis. This eliminates the manual effort involved in compiling reports from disparate sources, reducing the risk of errors and ensuring timely submission. Moreover, big data allows for more granular analysis of data, providing regulators with a clearer picture of an institution’s activities and risk profile.
Real-time dashboards can provide instant access to key compliance metrics, allowing institutions to monitor their performance and address any potential issues promptly.
Streamlining Regulatory Compliance Processes with Big Data
Regulation | Challenge | Big Data Solution | Implementation Details |
---|---|---|---|
AML | Identifying suspicious transactions amidst massive datasets | Machine learning algorithms for anomaly detection and network analysis | Integration with transaction processing systems, development of custom algorithms, ongoing model retraining |
KYC | Verifying customer identities and understanding business activities efficiently | Automated identity verification using diverse data sources, continuous monitoring of customer behavior | Integration with identity verification services, development of risk scoring models, implementation of real-time monitoring systems |
Regulatory Reporting | Aggregating data from various sources, ensuring accuracy and timeliness | Automated data aggregation and analysis, real-time dashboards for monitoring | Development of data pipelines, implementation of reporting automation tools, establishment of robust data governance frameworks |
Data Privacy | Ensuring compliance with data privacy regulations (e.g., GDPR, CCPA) | Data anonymization and encryption techniques, robust access control mechanisms | Implementation of data masking and tokenization, adoption of secure data storage solutions, development of comprehensive data privacy policies |
Algorithmic Trading and High-Frequency Trading
Big data is revolutionizing the financial markets, particularly in the realm of algorithmic and high-frequency trading (HFT). The sheer volume and velocity of data available today allow traders to develop sophisticated strategies that were previously unimaginable, leading to both significant gains and increased risks. This section explores the impact of big data on these trading methodologies.
Algorithmic trading, the use of computer programs to execute trades based on pre-defined rules, is heavily reliant on big data. The ability to process massive datasets in real-time allows algorithms to identify and exploit subtle patterns and anomalies that would be impossible for human traders to detect. This increased speed and efficiency translates directly into improved profitability and reduced transaction costs.
Big Data’s Influence on Algorithmic Trading Strategies
Big data provides algorithmic trading strategies with a significant advantage by enabling the analysis of vast quantities of information, including market data, news sentiment, social media trends, and economic indicators. This comprehensive data set allows algorithms to develop more sophisticated and adaptive trading strategies, leading to better predictions of market movements and more precise execution of trades. For instance, an algorithm might identify a correlation between specific news articles and subsequent price movements, allowing it to automatically buy or sell assets based on this correlation.
Examples of Big Data’s Use in Identifying Profitable Trading Opportunities
Several examples highlight how big data fuels profitable trading opportunities. One common application involves analyzing historical market data to identify recurring patterns or anomalies that predict future price movements. Another involves using alternative data sources, such as satellite imagery showing changes in parking lots near retail stores to gauge consumer demand and adjust investment strategies accordingly. Finally, sentiment analysis of social media posts and news articles can provide valuable insights into market sentiment, allowing algorithms to react quickly to shifts in investor confidence.
Big Data’s Role in High-Frequency Trading (HFT)
HFT, characterized by extremely rapid execution of large numbers of trades, is utterly dependent on big data. HFT algorithms require access to real-time market data with minimal latency to capitalize on fleeting price discrepancies. Big data infrastructure, including high-speed networks and powerful computing resources, is crucial for processing this data stream and making informed trading decisions within milliseconds.
This speed advantage allows HFT firms to profit from arbitrage opportunities and other short-term market inefficiencies.
Risks and Benefits of Using Big Data in Algorithmic Trading
While big data offers significant benefits to algorithmic trading, it also introduces new risks. The increased reliance on complex algorithms can lead to unforeseen consequences, such as algorithmic errors causing market disruptions or flash crashes. Additionally, the potential for data bias and manipulation poses a significant threat. However, the benefits, such as improved efficiency, increased profitability, and reduced transaction costs, often outweigh these risks when appropriate safeguards and risk management strategies are implemented.
Data Flow in Algorithmic Trading
Imagine a data flow diagram starting with diverse data sources: market data feeds (prices, volumes, order books), news feeds (financial news, social media sentiment), economic indicators (GDP, inflation), and alternative data (satellite imagery, credit card transactions). This raw data is then ingested and cleaned, undergoing transformation and normalization. Next, the data is fed into sophisticated algorithms that employ machine learning techniques to identify patterns and predict future price movements.
The algorithm’s output—buy or sell signals—is then routed to an order management system that executes trades on exchanges. Finally, the results of these trades, along with new data, are fed back into the system, creating a continuous feedback loop that constantly refines the algorithm’s performance. This cyclical process ensures continuous adaptation to changing market conditions.
Enhanced Customer Service and Support: How Big Data Is Transforming The Financial Services Industry And Risk Management
Big data analytics is revolutionizing customer service in the financial sector, moving beyond reactive support to a proactive, personalized experience. By leveraging vast datasets encompassing customer interactions, transaction history, and market trends, financial institutions can anticipate needs, resolve issues more efficiently, and build stronger customer relationships. This results in increased customer satisfaction, reduced operational costs, and improved brand loyalty.Big data empowers financial institutions to understand their customers on a deeper level than ever before.
Analyzing customer data reveals patterns and preferences, allowing for the development of targeted support strategies and the creation of personalized financial products and services. This shift towards data-driven customer service is transforming the industry, offering a competitive advantage to those who effectively harness its power.
Chatbots and Virtual Assistants Powered by Big Data
Big data fuels the development of sophisticated chatbots and virtual assistants capable of handling a wide range of customer inquiries. These AI-powered tools leverage natural language processing (NLP) and machine learning (ML) algorithms trained on extensive datasets of customer interactions. For example, a chatbot might be trained on millions of past customer service tickets to identify common issues and provide instant solutions.
This allows for 24/7 availability, immediate responses, and a consistent customer experience across all channels. Furthermore, advanced chatbots can analyze customer sentiment and adapt their responses accordingly, improving customer satisfaction and reducing frustration. A well-trained chatbot can handle routine tasks such as account balance inquiries, transaction confirmations, and password resets, freeing up human agents to focus on more complex issues.
Proactive Customer Support Through Big Data Analysis
Big data enables proactive customer support by identifying potential problems before they arise. By analyzing transaction data, customer behavior patterns, and market trends, financial institutions can anticipate potential risks and proactively reach out to customers. For example, if the system detects unusual spending patterns on a customer’s credit card, it can send an alert, preventing potential fraud. Similarly, if a customer’s investment portfolio is underperforming compared to their risk profile, the system can trigger a recommendation for portfolio adjustments.
This proactive approach fosters trust and loyalty, demonstrating the institution’s commitment to customer well-being. Predictive modeling based on big data can forecast customer churn, allowing for timely intervention and retention strategies.
Efficiency Comparison: Big Data vs. Traditional Customer Support
Traditional customer support methods often rely on reactive responses to customer inquiries, leading to longer wait times, increased operational costs, and potentially dissatisfied customers. Big data-driven customer support, however, offers significantly improved efficiency. Automated responses to routine inquiries through chatbots and virtual assistants reduce the workload on human agents, allowing them to focus on more complex issues requiring specialized knowledge.
Proactive interventions prevent problems from escalating, minimizing the need for reactive support. Data-driven insights allow for better resource allocation, optimizing staffing levels and reducing operational costs. The overall result is a more efficient and cost-effective customer support system. For instance, a large bank might see a 30% reduction in call center wait times and a 15% decrease in customer service costs after implementing a big data-powered support system.
System Architecture for a Big Data-Powered Customer Support Platform
A robust big data-powered customer support platform requires a well-designed architecture. This architecture would typically include: (1) Data Ingestion: A system for collecting data from various sources, including CRM systems, transaction databases, social media, and customer service interactions. (2) Data Storage: A distributed storage system like Hadoop Distributed File System (HDFS) or cloud-based object storage to handle the massive volume of data.
(3) Data Processing: A real-time processing engine like Apache Spark or Apache Kafka to analyze data and generate insights. (4) Machine Learning Models: Algorithms for natural language processing, sentiment analysis, predictive modeling, and anomaly detection. (5) Customer Interaction Channels: Integration with various channels such as chatbots, virtual assistants, email, phone, and social media. (6) Reporting and Analytics Dashboard: A user-friendly interface for visualizing data, monitoring performance, and identifying areas for improvement.
This comprehensive architecture ensures efficient data processing, real-time insights, and seamless integration with various customer service channels.